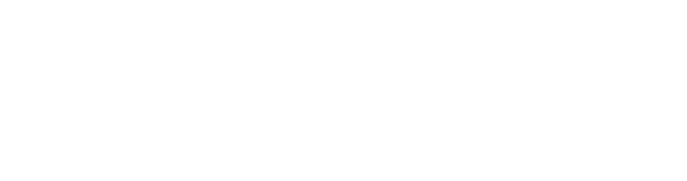
Purchase your New Stata 19 Student License here, with rapid downloads sent directly to your inbox.
Complete Your Order Here
Choose the number of licenses, license term and the product you are after and add to basket.
Navigate to 'compare versions' below to discover more about each flavour of Stata. Still need help? Contact us to discuss your requirements.
Navigate here to purchase, lab licenses start at 10 users.
- Comparer les versions
- Dernières fonctionnalités
- System Requirements
- Présentation du logiciel
Comparer Stata
Stata est une suite logicielle complète et intégrée qui répond à tous vos besoins en science des données : manipulation, visualisation, statistiques et reporting automatisé. Stata n'est pas vendu sous forme de modules, ce qui signifie que vous disposez de tout ce dont vous avez besoin dans une seule et même solution.
Que vous soyez étudiant ou professionnel de la recherche chevronné, une gamme de packages Stata est disponible et conçue pour répondre à tous les besoins.
Toutes les éditions suivantes de Stata disposent du même ensemble complet de commandes et de fonctionnalités, ainsi que de manuels inclus sous forme de documentation PDF dans Stata.
- Stata/MP : l'édition la plus rapide de Stata (pour les ordinateurs à quatre cœurs, à deux cœurs et multicœurs/multiprocesseurs) qui peut analyser les plus grands ensembles de données
- Stata/SE (édition standard) : édition standard ; pour les ensembles de données plus volumineux
- Stata/BE (Basic Edition) : édition de base ; pour les ensembles de données de taille moyenne
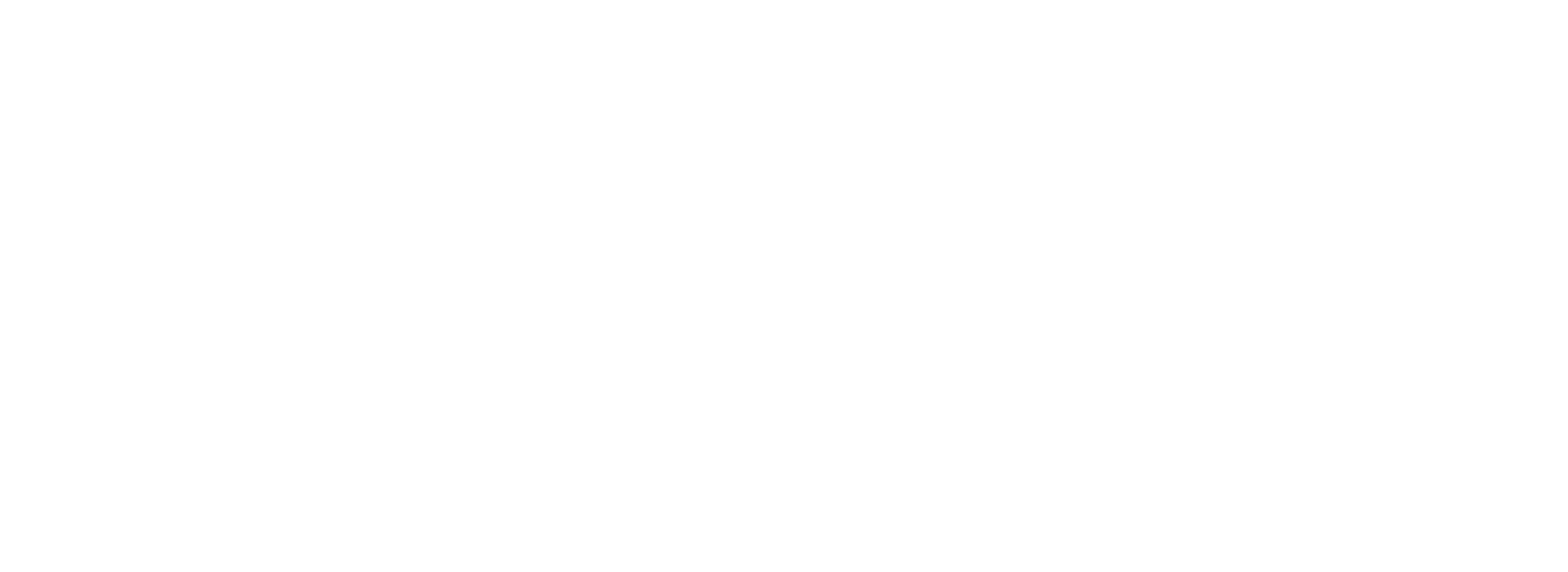
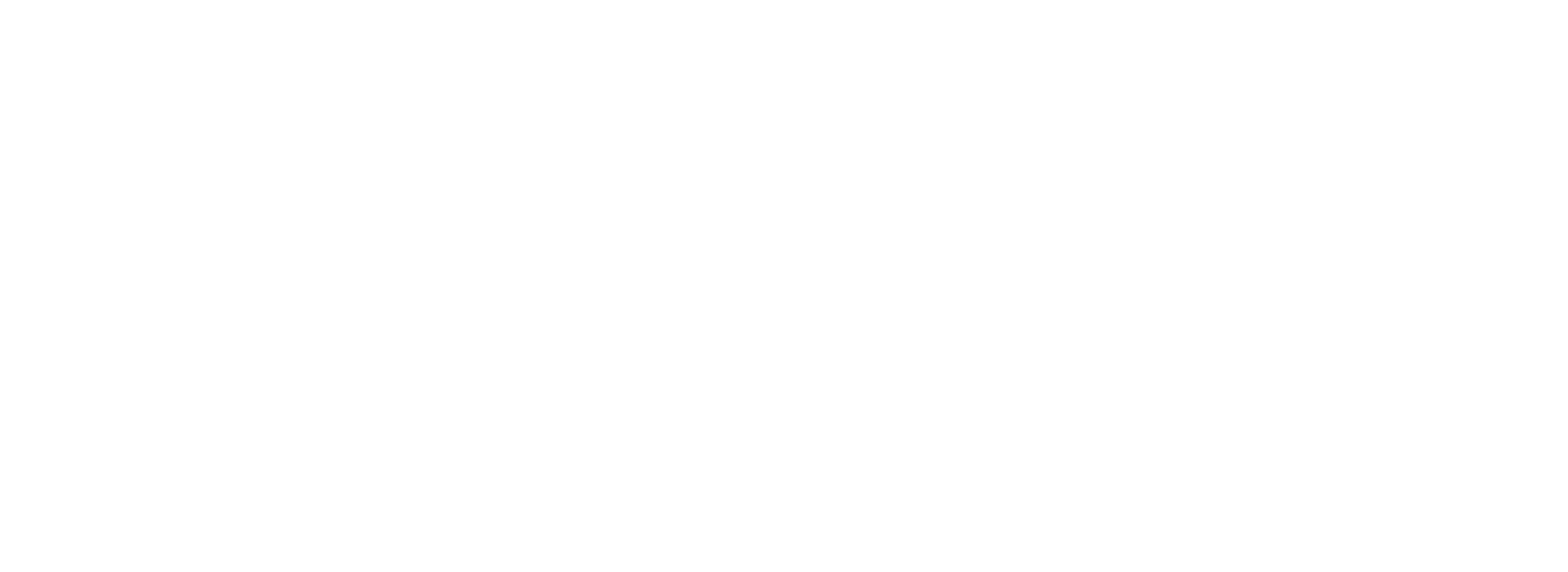
Stata/MP est l'édition la plus rapide et la plus complète de Stata. Pratiquement tous les ordinateurs actuels peuvent bénéficier du multitraitement avancé de Stata/MP. Cela inclut les processeurs Intel i3, i5, i7, i9, Xeon et Celeron, ainsi que les puces multicœurs AMD. Sur les puces double cœur, Stata/MP est globalement 40 % plus rapide et 72 % plus rapide là où c'est nécessaire, sur les commandes d'estimation chronophages. Avec plus de deux cœurs ou processeurs, Stata/MP est encore plus rapide.
Stata/MP est plus rapide, bien plus rapide. Stata/MP vous permet d'analyser vos données deux fois plus vite, voire deux fois plus vite que Stata/SE sur les ordinateurs portables bicœurs bon marché, et quatre fois plus vite, voire deux fois plus vite, sur les ordinateurs portables et de bureau quaternaires.
Stata/MP est encore plus rapide sur les serveurs multiprocesseurs. Stata/MP prend en charge jusqu'à 64 processeurs/cœurs.
La vitesse est souvent un critère crucial lors de l'exécution de procédures d'estimation exigeantes en calculs. Certaines procédures d'estimation de Stata, notamment la régression linéaire, sont presque parfaitement parallélisées : elles s'exécutent deux fois plus vite sur deux cœurs, quatre fois plus vite sur quatre cœurs, huit fois plus vite sur huit cœurs, etc. Certaines commandes d'estimation sont plus parallélisables que d'autres. En moyenne, les commandes d'estimation s'exécutent 1,8 fois plus vite sur deux cœurs, 2,9 fois plus vite sur quatre cœurs et 4,1 fois plus vite sur huit cœurs.
Stata/MP est entièrement compatible avec les autres éditions de Stata. Les analyses n'ont pas besoin d'être reformulées ou modifiées pour bénéficier des améliorations de vitesse de Stata/MP.
Stata/MP est disponible pour les systèmes d'exploitation suivants :
- Windows (processeurs 64 bits) ;
- macOS (processeurs Intel 64 bits) ;
- Linux (processeurs 64 bits) ;
Pour exécuter Stata/MP, vous pouvez utiliser un ordinateur de bureau équipé d'un processeur double ou quadruple cœur, ou un serveur multiprocesseur. Qu'un ordinateur soit équipé de processeurs distincts ou d'un processeur multicœur n'a aucune importance. Plus il y a de processeurs ou de cœurs, plus Stata/MP est rapide.
Pour plus de conseils sur l'achat/la mise à niveau vers Stata/MP ou pour des questions sur le matériel, veuillez contacter notre équipe commerciale.
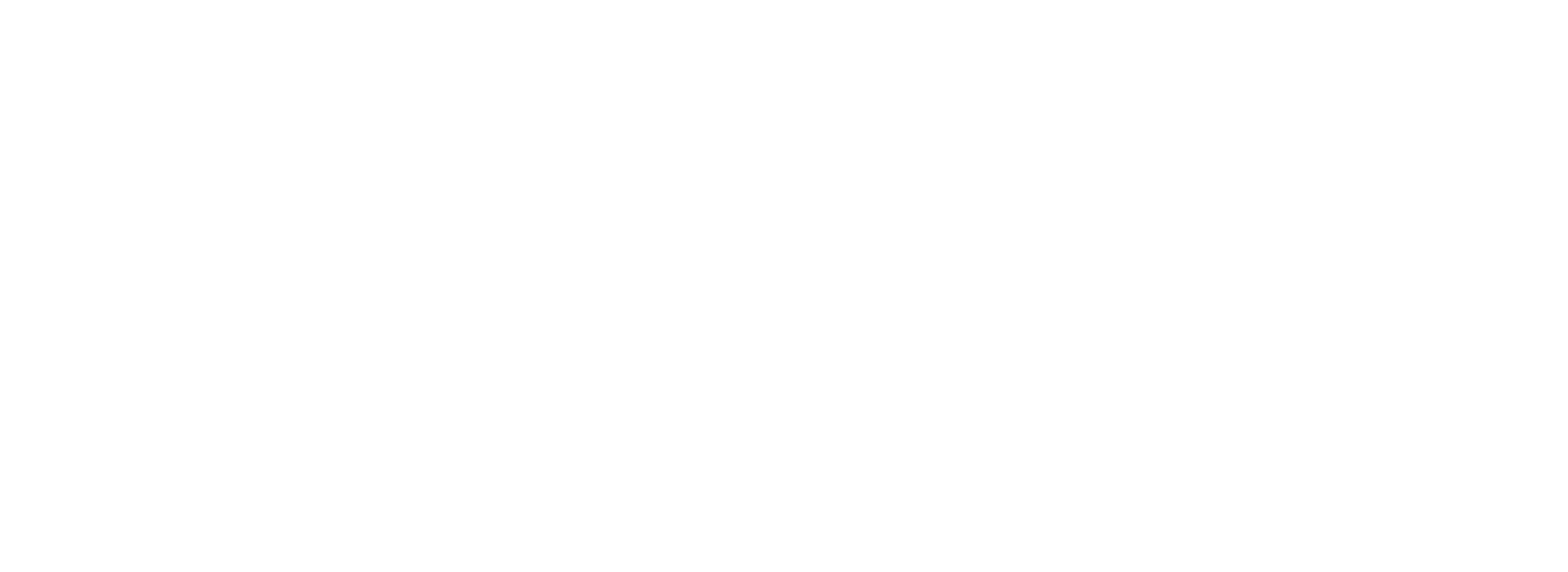
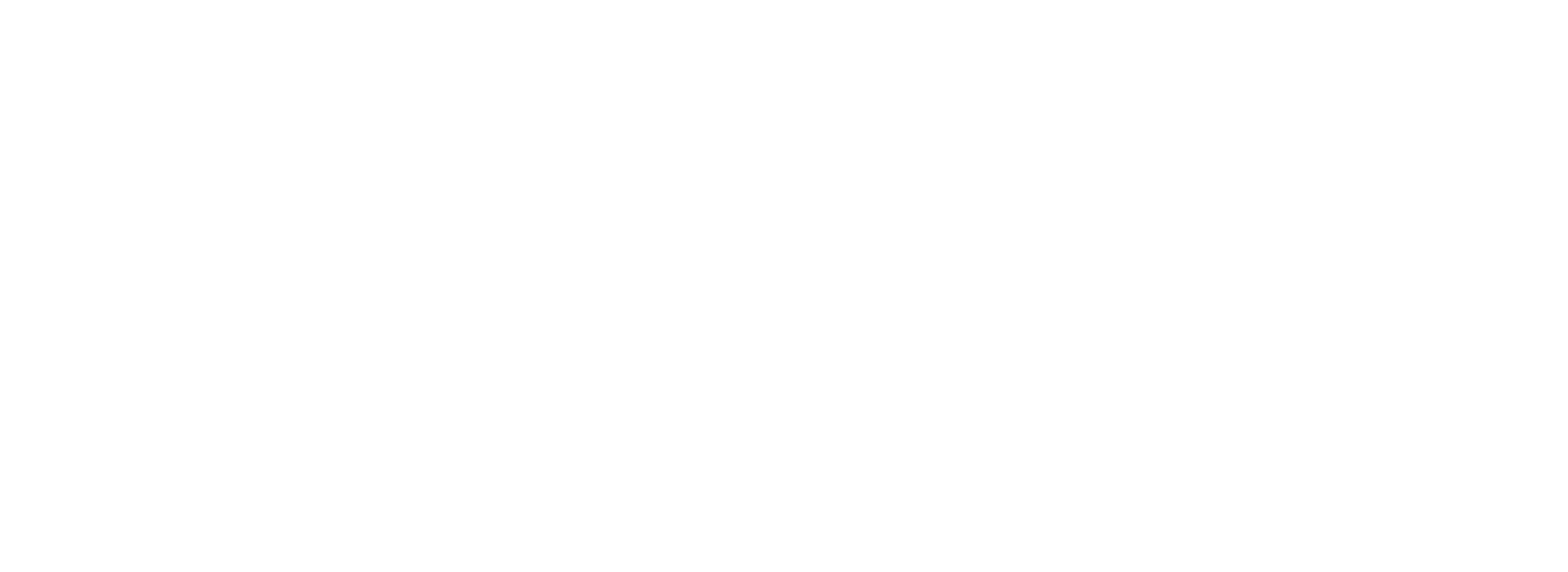
Stata/SE et Stata/BE ne diffèrent que par la taille de l'ensemble de données qu'ils peuvent analyser. Stata/SE et Stata/MP peuvent ajuster des modèles avec davantage de variables indépendantes que Stata/BE (jusqu'à 65 532). Stata/SE peut analyser jusqu'à 2 milliards d'observations.
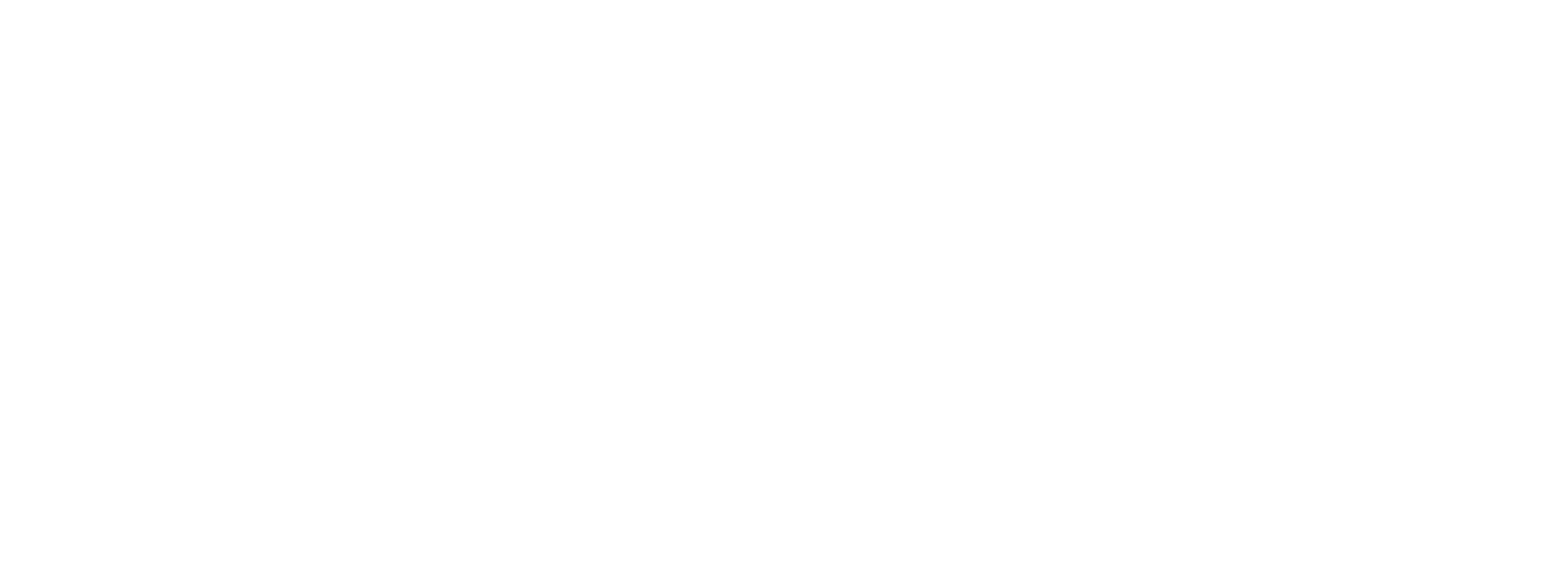
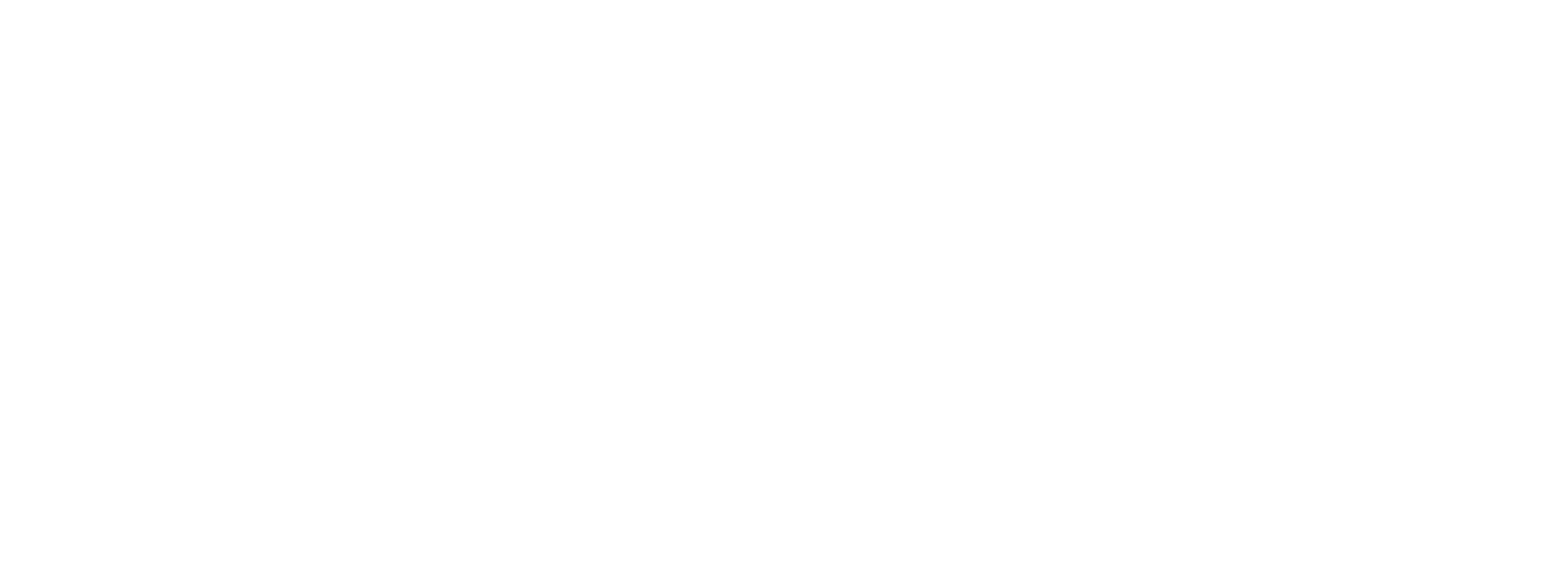
Stata/BE autorise des jeux de données contenant jusqu'à 2 048 variables. Le nombre maximal d'observations est de 2,14 milliards. Stata/BE peut contenir jusqu'à 798 variables indépendantes dans un modèle.
Product Features | Stata/BE | Stata/SE | Stata/MP |
---|---|---|---|
Maximum number of variables Up to 2,048 Variables Up to 32,767 variables Up to 120,000 variables |
|
|
|
Maximum number of observations Up to 2.14 billion Up to 20 billion |
|
|
|
Speed Comparisons Fast Twice as fast
|
|
|
|
Time to run logistic regression with 10 million observations and 20 covariates 20 seconds 10 seconds |
|
|
|
Complete suite of statistical features |
![]() |
![]() |
![]() |
Publication-quality graphics |
|
![]() |
![]() |
Extensive data management facilities |
|
|
![]() |
Truly reproducible research |
|
|
![]() |
Comprehensive reporting and table generation |
|
|
![]() |
Powerful programming language |
|
![]() |
![]() |
Complete PDF documentation |
|
![]() |
![]() |
Exceptional technical support |
|
![]() |
![]() |
Includes within-release updates through StataNow |
|
![]() |
![]() |
Windows, macOS and Linux |
|
|
|
And much more for all your data science needs |
|
|
|
Memory requirements |
1GB | 2GB | 4GB |
Disk space requirements |
2GB | 2GB | 2GB |
Quoi de neuf dans Stata 19
Approfondissez vos recherches avec les dernières fonctionnalités de Stata 19.
Stata 19 a quelque chose à offrir à chacun. Nous présentons ci-dessous les points forts de cette version. Stata 19 est unique car la plupart des nouvelles fonctionnalités sont accessibles aux chercheurs de toutes disciplines.
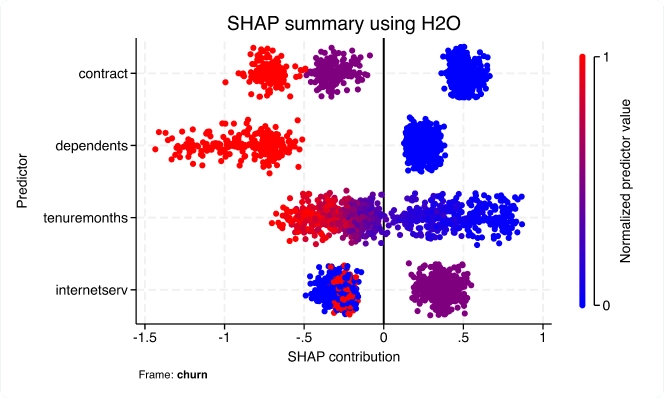
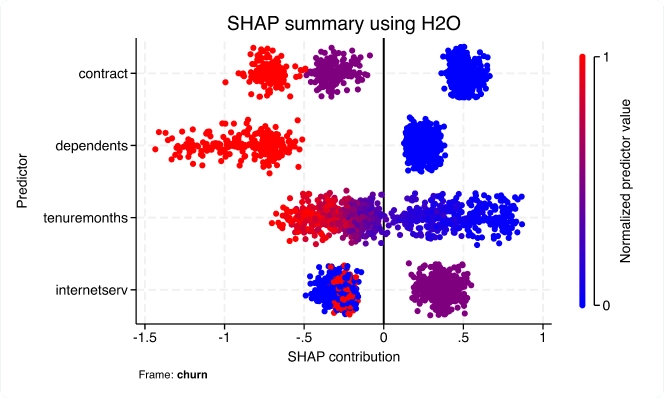
Apprentissage automatique via H2O : arbres de décision d'ensemble
Avec la nouvelle suite h2oml, utilisez le machine learning via H2O pour extraire des informations pertinentes de vos données lorsque les modèles statistiques traditionnels sont insuffisants. Les méthodes de machine learning sont souvent utilisées pour résoudre des problèmes de recherche et d'entreprise axés sur la prédiction.
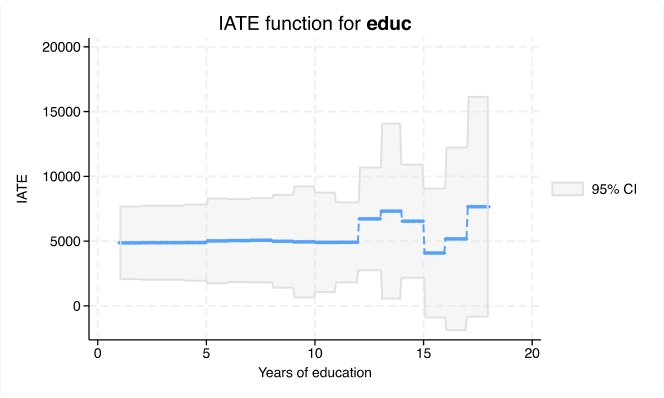
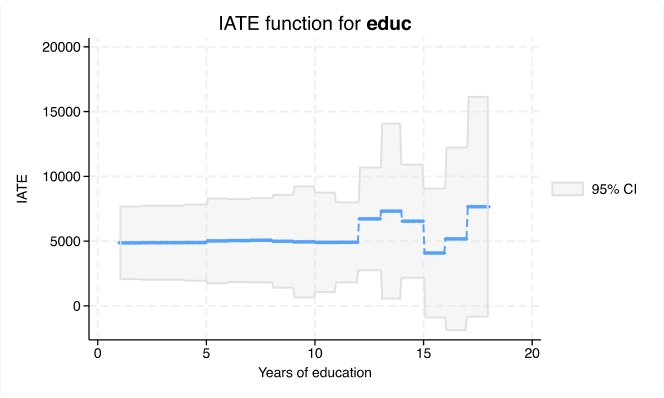
Effets moyens conditionnels du traitement (CATE)
Avec la nouvelle commande cate, vous pouvez aller au-delà de l’estimation d’un effet de traitement global pour estimer des effets individualisés ou spécifiques à un groupe qui répondent à ces types de questions de recherche.
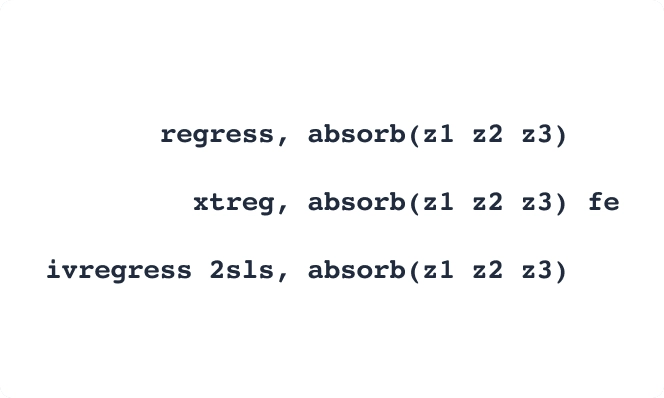
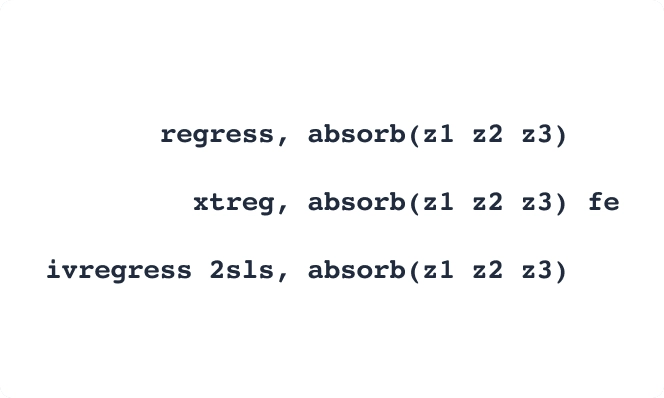
Effets fixes haute dimension (HDFE)
Absorbez non pas une mais plusieurs variables catégorielles de grande dimension dans vos modèles linéaires et à effets fixes avec l'option absorb() des commandes areg et xtreg.
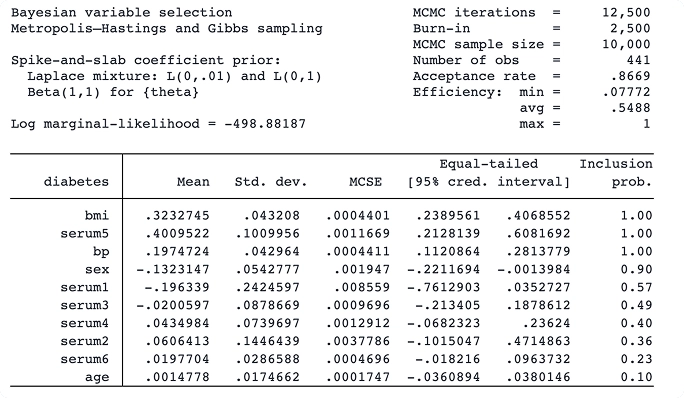
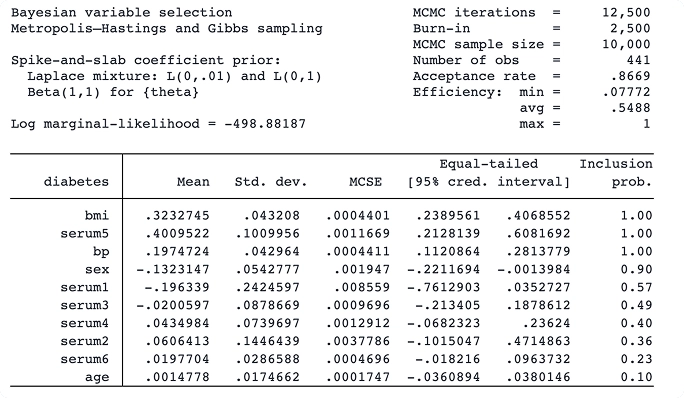
Sélection de variables bayésiennes pour la régression linéaire
Grâce à la nouvelle commande bayesselect, vous pouvez effectuer une sélection bayésienne de variables pour la régression linéaire. Cette approche offre une interprétation intuitive et une inférence stable, tenant compte de l'incertitude du modèle.
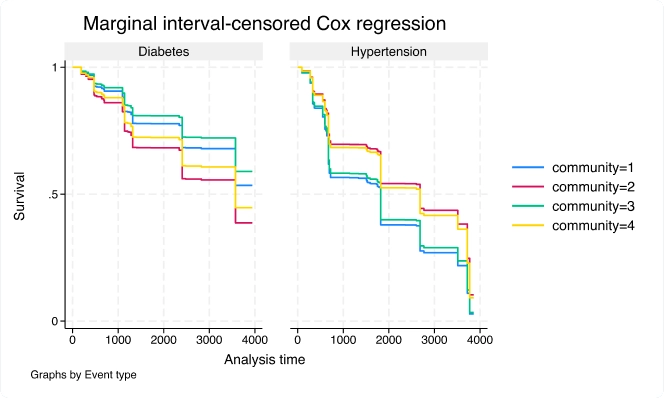
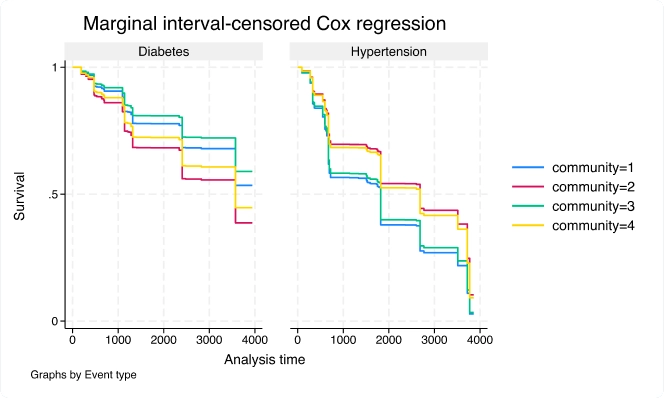
Modèles marginaux de Cox PH pour les données d'événements multiples censurées par intervalle
Utilisez la nouvelle commande stmgintcox pour analyser les données d’événements multiples censurées par intervalle.
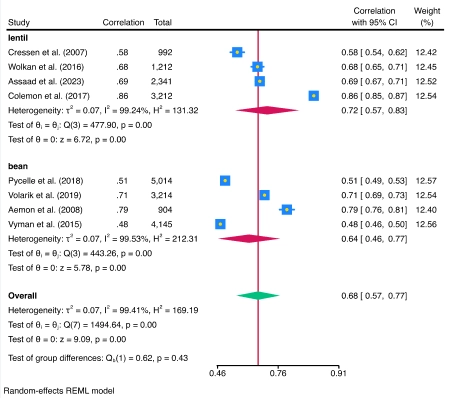
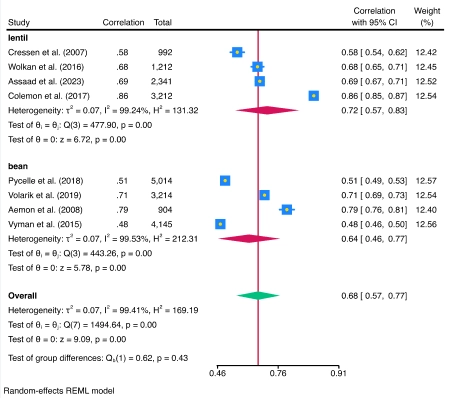
Méta-analyse des corrélations
La suite méta prend désormais en charge la méta-analyse (MA) d'un coefficient de corrélation. Toutes les fonctionnalités standard de méta-analyse, telles que les graphiques en forêt et l'analyse de sous-groupes, sont prises en charge.
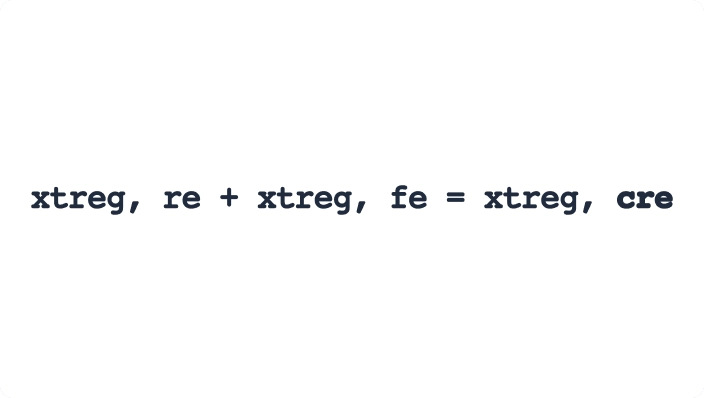
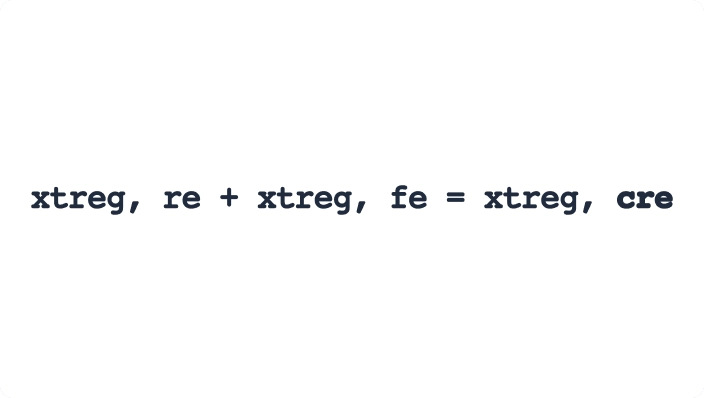
Modèle à effets aléatoires corrélés (CRE)
Vous souhaitez estimer les coefficients des covariables invariantes dans le temps dans votre modèle de données de panel ? Avec xtreg, cre , vous pouvez désormais ajuster un modèle à effets aléatoires corrélés.
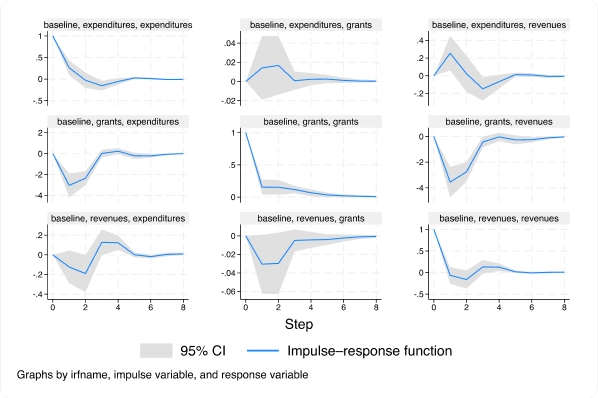
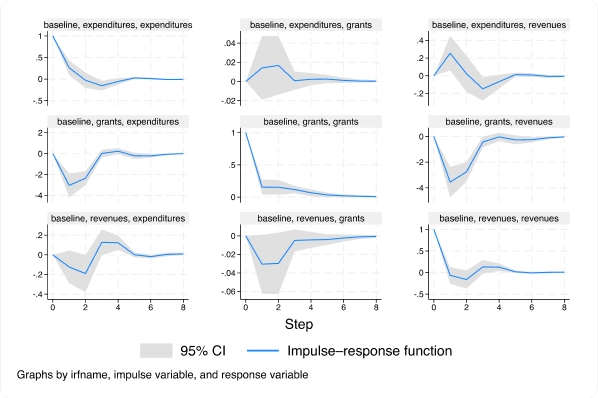
Modèle vectoriel autorégressif (VAR) basé sur des données de panel
Avec la nouvelle commande xtvar, vous pouvez désormais ajuster un modèle vectoriel autorégressif (VAR) de données de panel pour analyser les trajectoires de variables associées lorsque vous observez plusieurs unités ou panneaux au fil du temps.
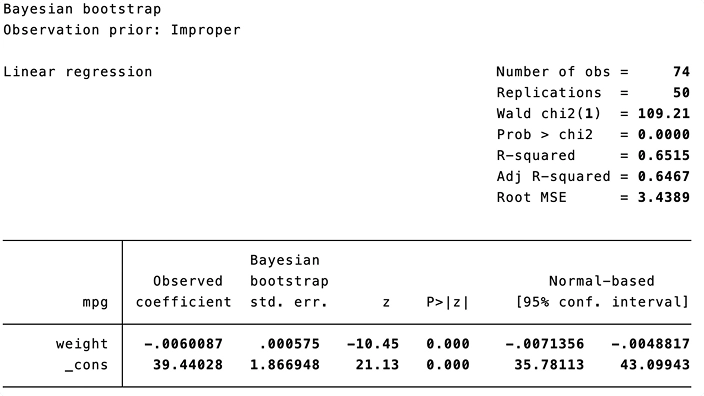
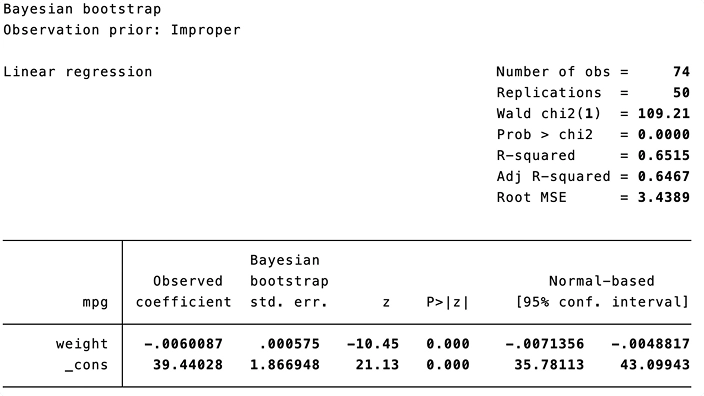
Bootstrap bayésien et pondérations répliquées
Vous pouvez utiliser le nouveau préfixe bayesboot pour effectuer un bootstrap bayésien des statistiques produites par les commandes officielles et celles de la communauté. Le bootstrap bayésien peut intégrer des informations a priori pour obtenir des estimations de paramètres plus précises.
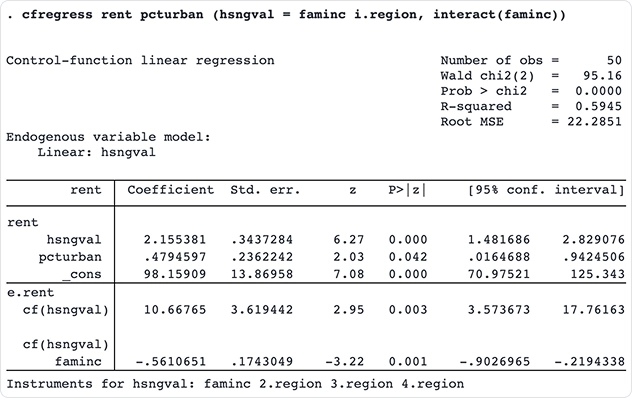
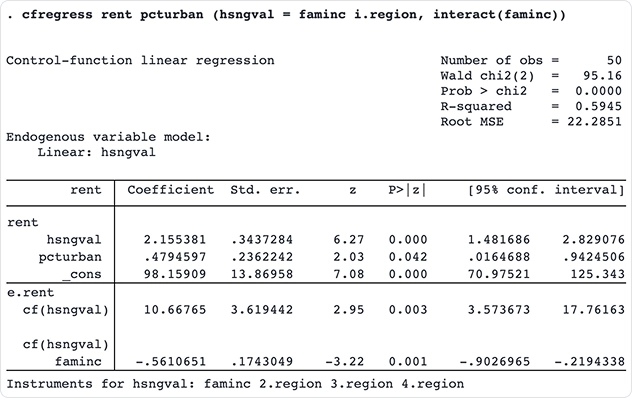
Modèles linéaires et probit à fonction de contrôle
Ajustez les modèles linéaires et probits à fonction de contrôle avec les nouvelles commandes cfregress et cfprobit. Les modèles à fonction de contrôle offrent une approche plus flexible que les méthodes traditionnelles à variables instrumentales (VI) en incluant des variables endogènes.
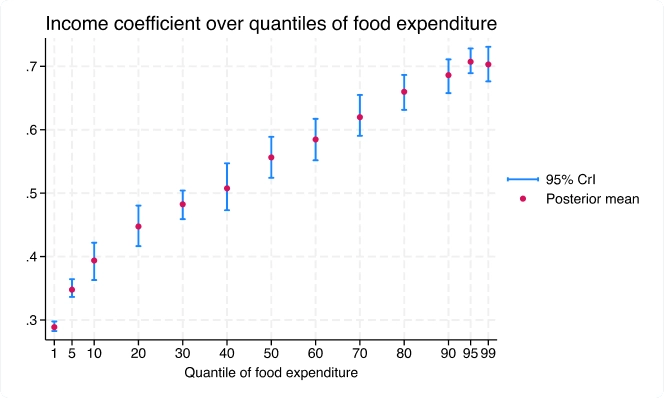
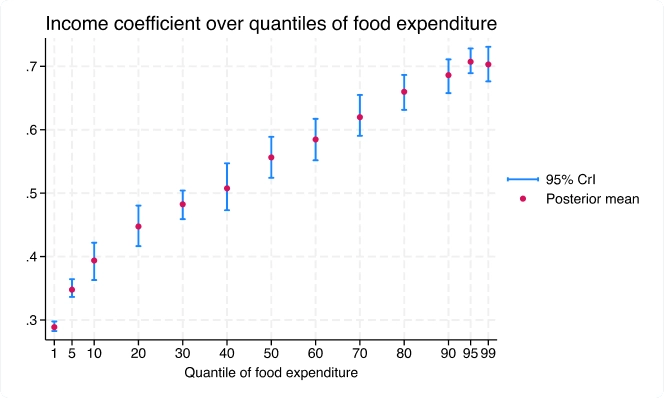
Régression quantile bayésienne via la vraisemblance asymétrique de Laplace
La nouvelle commande bayes:qreg s'adapte à la régression quantile bayésienne. Le cadre bayésien fournit des distributions postérieures complètes pour les coefficients de régression quantile, offrant ainsi une inférence complète.
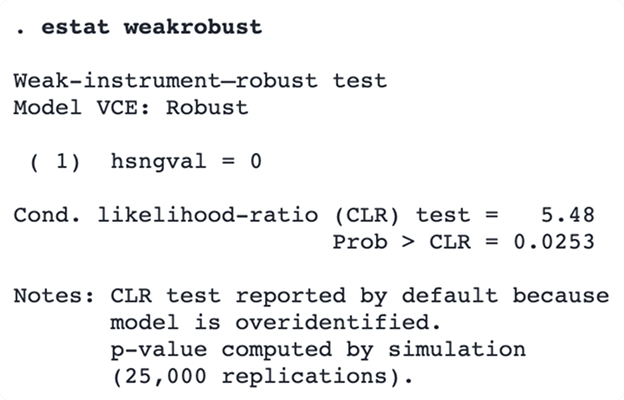
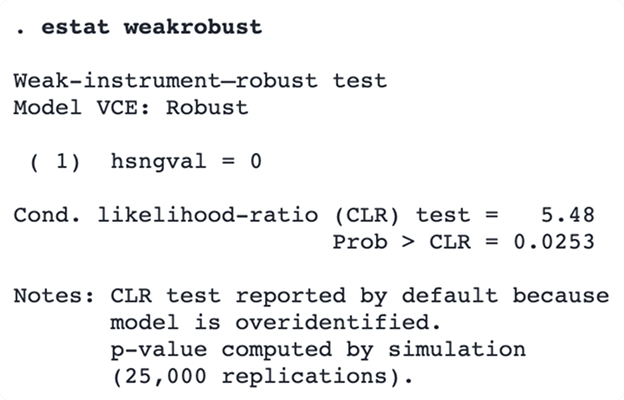
Inférence robuste aux instruments faibles
Utilisez la nouvelle commande estat weakrobust pour effectuer une inférence fiable sur les régresseurs endogènes.
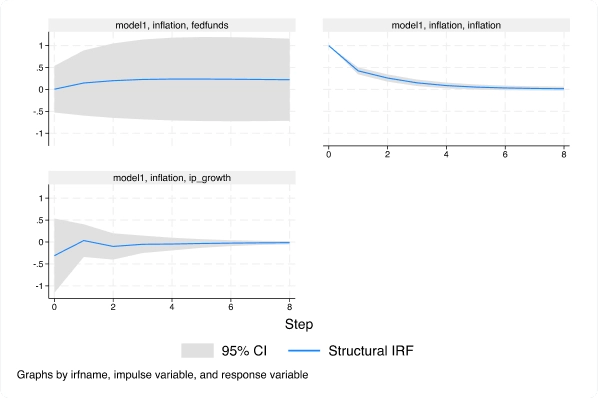
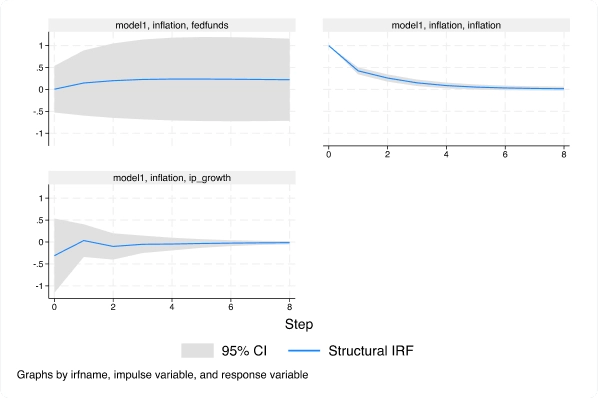
Modèles vectoriels autorégressifs structurels (SVAR) via des variables instrumentales
Avec la nouvelle commande ivsvar, vous pouvez utiliser des instruments au lieu de contraintes à court terme pour estimer les effets causaux dynamiques.
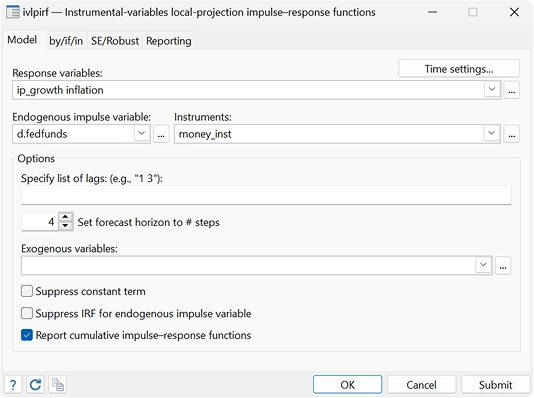
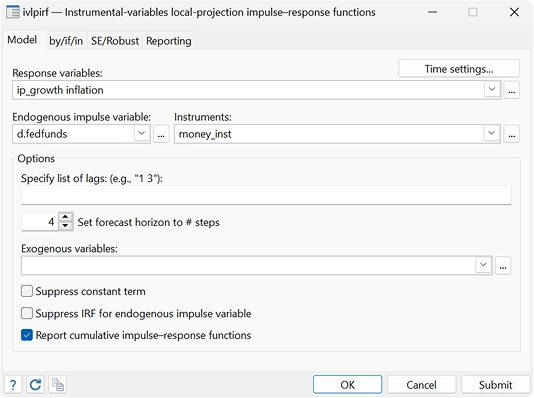
FRI à projection locale à variables instrumentales
Avec la nouvelle commande ivlpirf, vous pouvez prendre en compte l’endogénéité lors de l’utilisation de projections locales pour estimer les effets causaux dynamiques.
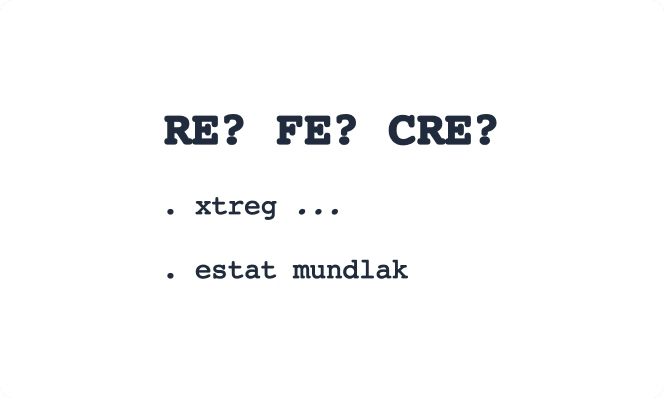
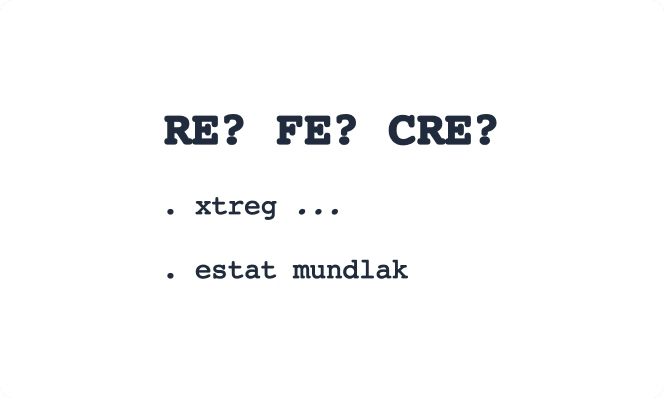
Test de spécification Mundlak
Utilisez la nouvelle commande de post-estimation estat mundlak après xtreg pour choisir entre des modèles à effets aléatoires (RE), à effets fixes (FE) ou à effets aléatoires corrélés (CRE), même avec des erreurs standard robustes en cluster, bootstrap ou jackknife.


Statistiques de comparaison de modèles de classes latentes
Avec la nouvelle commande lcstats n, vous pouvez utiliser des statistiques telles que l'entropie et une variété de critères d'information pour vous aider à déterminer le nombre approprié de classes.
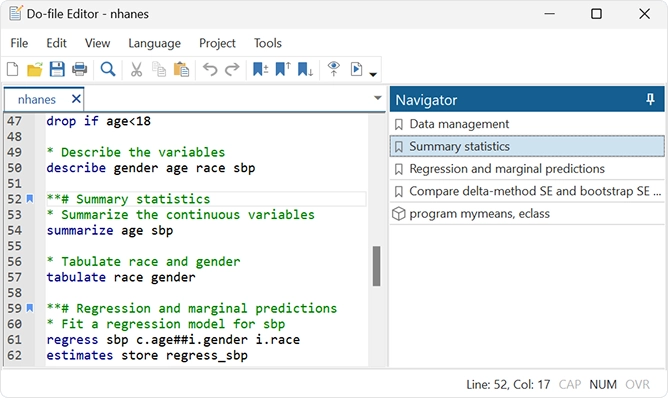
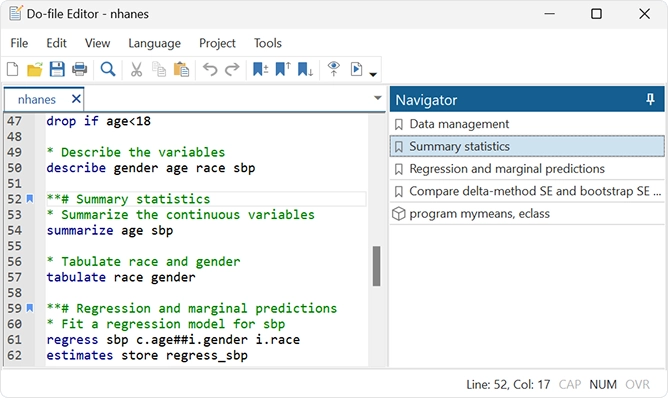
Éditeur Do-file : saisie semi-automatique, modèles et plus encore
L'éditeur Do-file présente les ajouts suivants : saisie semi-automatique des noms de variables, des macros et des résultats stockés ; améliorations du pliage de code ; signets temporaires et permanents ; modèles, onglets et panneau de navigation.
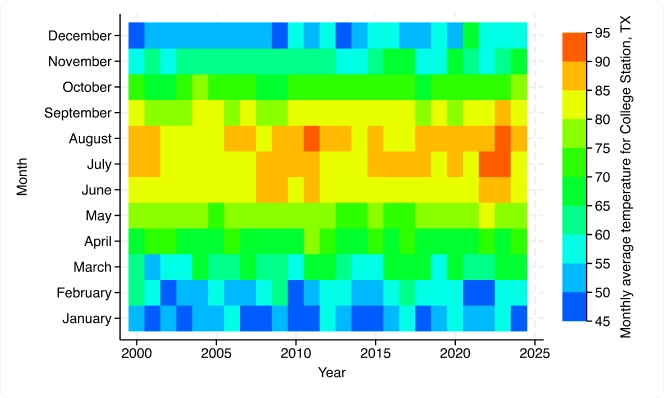
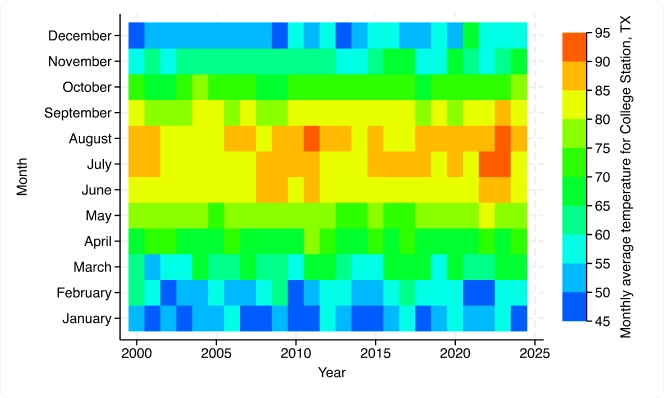
Graphiques : graphiques à barres CI, cartes thermiques et plus encore
Nouvelles fonctionnalités graphiques : Cartes thermiques (bidirectionnelles) ; Tracé de plage et de points avec pics plafonnés (bidirectionnelles) ; Tracé de plage et de points avec pics (bidirectionnelles) ; Étiquetage amélioré, CI et contrôle des regroupements pour les graphiques à barres, les graphiques à points et les boîtes à moustaches ; Couleurs par variable pour plus de graphiques.
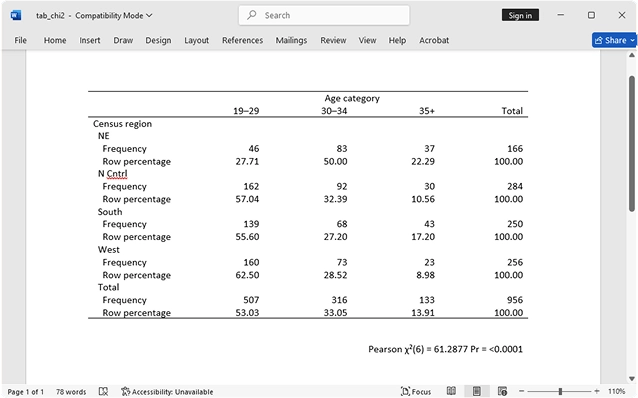
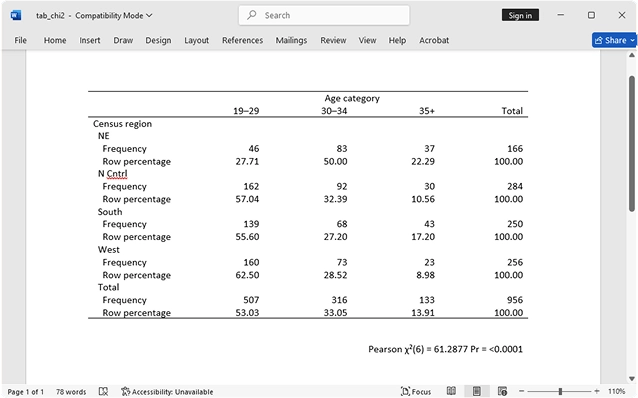
Tableaux : tabulations, exportations et bien plus encore plus faciles
Créez et personnalisez facilement des tableaux avec des titres, des notes et des exportations. La commande « tableau » est un outil flexible permettant de créer des tabulations, des tableaux de statistiques récapitulatives, des tableaux de résultats de régression, etc.
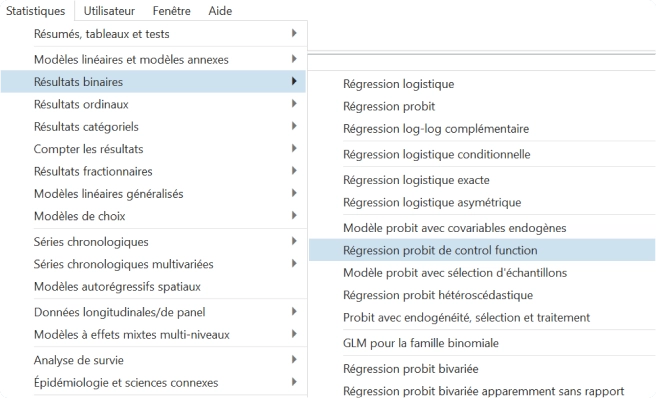
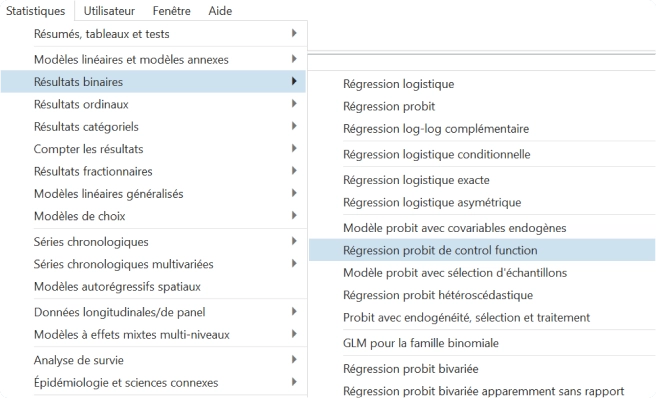
Stata en français
Les menus, boîtes de dialogue et autres éléments de Stata peuvent désormais être affichés en français. Si la langue de votre ordinateur est définie sur le français (fr), Stata utilisera automatiquement le paramètre français.
System Requirements
OS | Windows 10 Macs with Apple Silicon and macOS 10.13 or newer for Macs with Intel processors |
---|---|
Processor | Applie Silicon, Intel or AMD processor (Core i3 equivalent or better) |
Memory | Stata/MP > 4GB, Stata/SE > 2GB, and Stata/BE 1GB |
Hard Drive | 4GB |
Pourquoi Stata ?
Rapide. Précis. Facile à utiliser. Stata est un logiciel complet et intégré qui répond à tous vos besoins en science des données : manipulation, visualisation, statistiques et reporting automatisé.
|
|
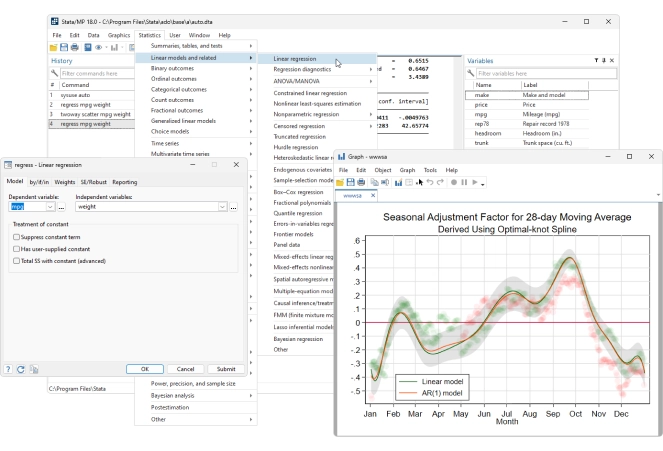
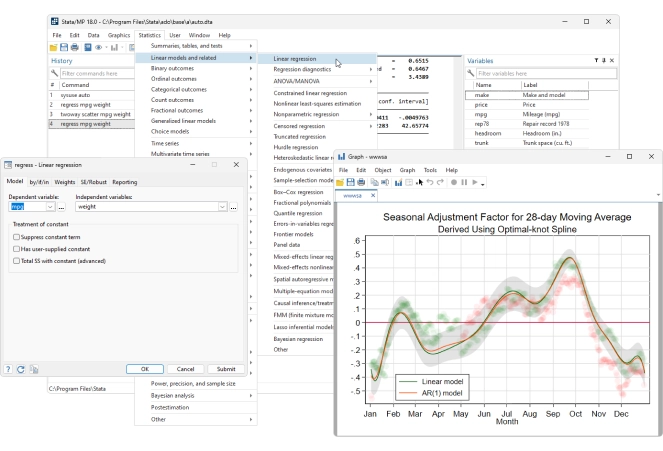
Maîtrisez vos données
Les fonctionnalités de gestion des données de Stata vous offrent un contrôle total.
|
|
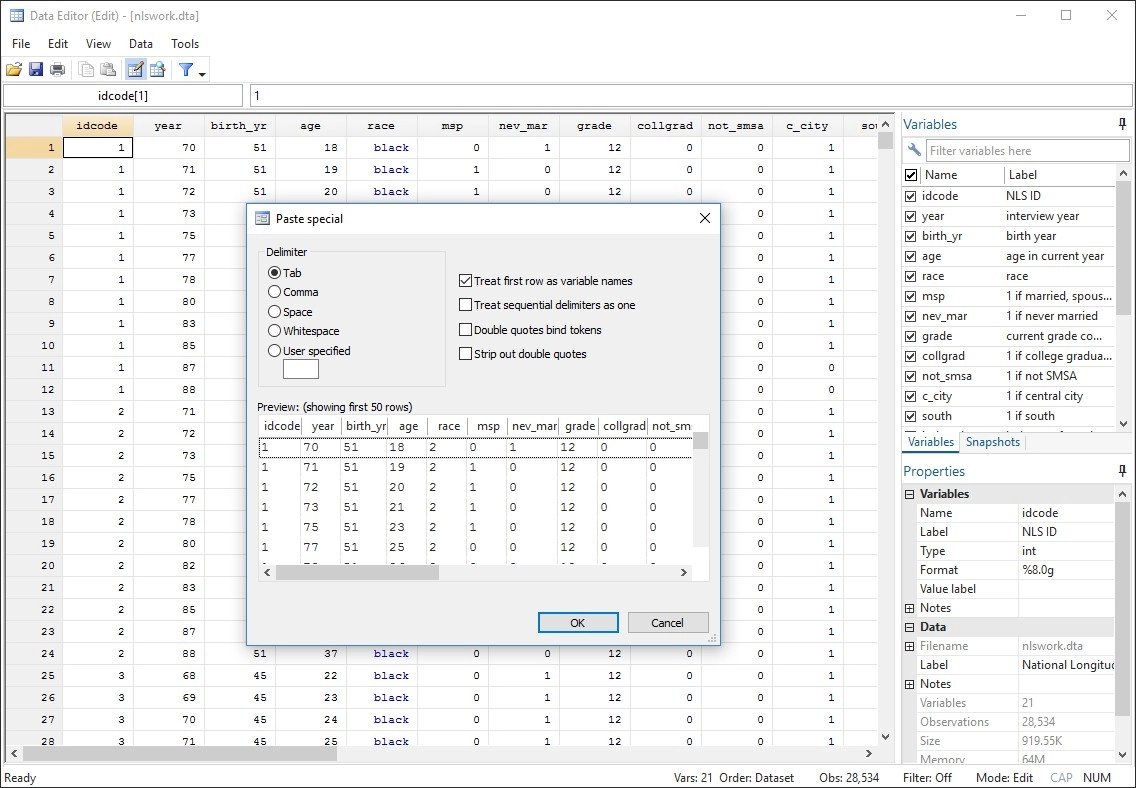
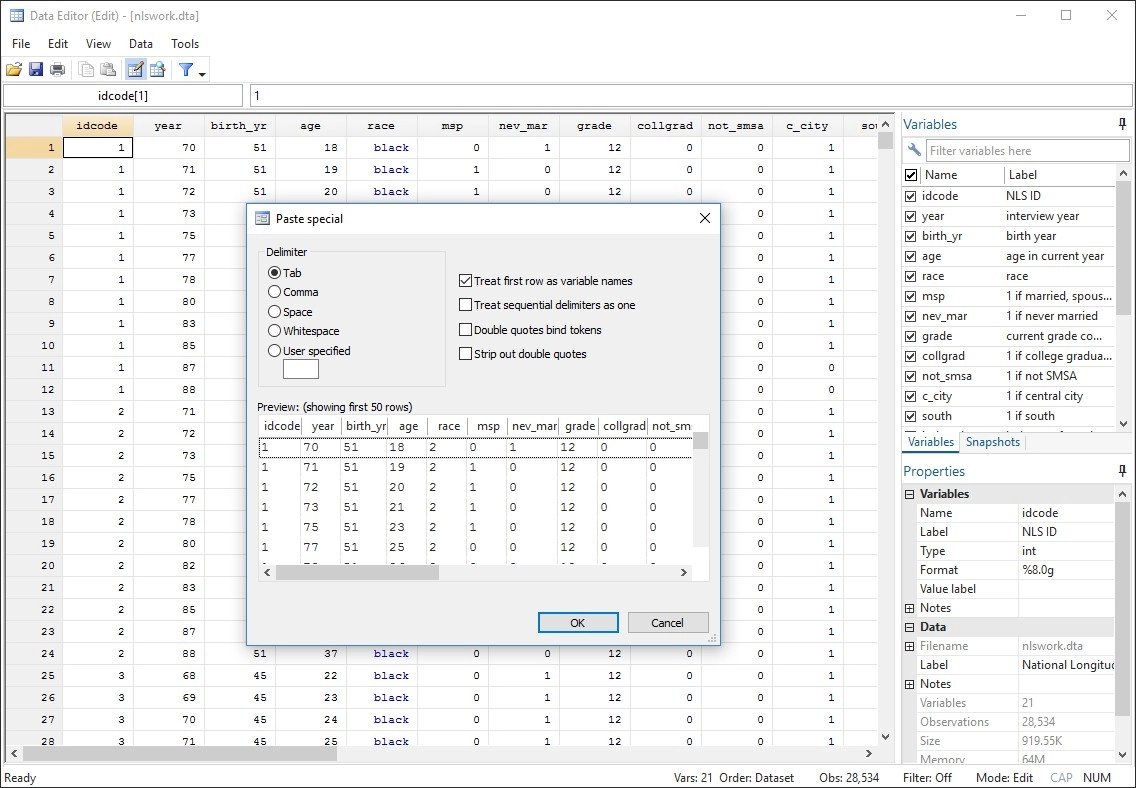
Graphiques de qualité des publications
Stata facilite la génération de graphiques de qualité publication, au style distinctif.
Vous pouvez pointer et cliquer pour créer un graphique personnalisé. Vous pouvez également écrire des scripts pour générer des centaines, voire des milliers de graphiques.
de manière reproductible.
Exportez des graphiques au format EPS ou TIFF pour publication, au format PNG ou SVG pour le Web ou au format PDF pour visualisation.
Avec l'éditeur de graphiques intégré, vous cliquez pour modifier quoi que ce soit sur votre graphique ou pour ajouter des titres, des notes, des lignes, des flèches et du texte.
Rapports automatisés
Tous les outils dont vous avez besoin pour automatiser le reporting de vos résultats.
- Document Markdown dynamique
- Créer des documents Word
- Créer des documents PDF
- Créer des fichiers Excel
- Tables personnalisables
- Schémas pour les graphiques
- Word, HTML, PDF, SVG, PNG
PyStata - Intégration Python
Invoquez Python de manière interactive ou intégrez Python dans votre code Stata.
Appelez Stata depuis Python et appelez le code Stata depuis les environnements IPython.
Utilisez Stata dans Jupyter Notebook.
Transmettez de manière transparente les données et les résultats entre Stata et Python.
Utilisez les analyses Stata depuis Python.
Utilisez n'importe quel package Python dans Stata
- Matplotlib et Seaborn pour la visualisation
- Belle soupe et Scrapy pour le web scraping
- NumPy et pandas pour l'analyse numérique
- TensorFlow et scikit-learn pour l'apprentissage automatique
- Et bien plus encore
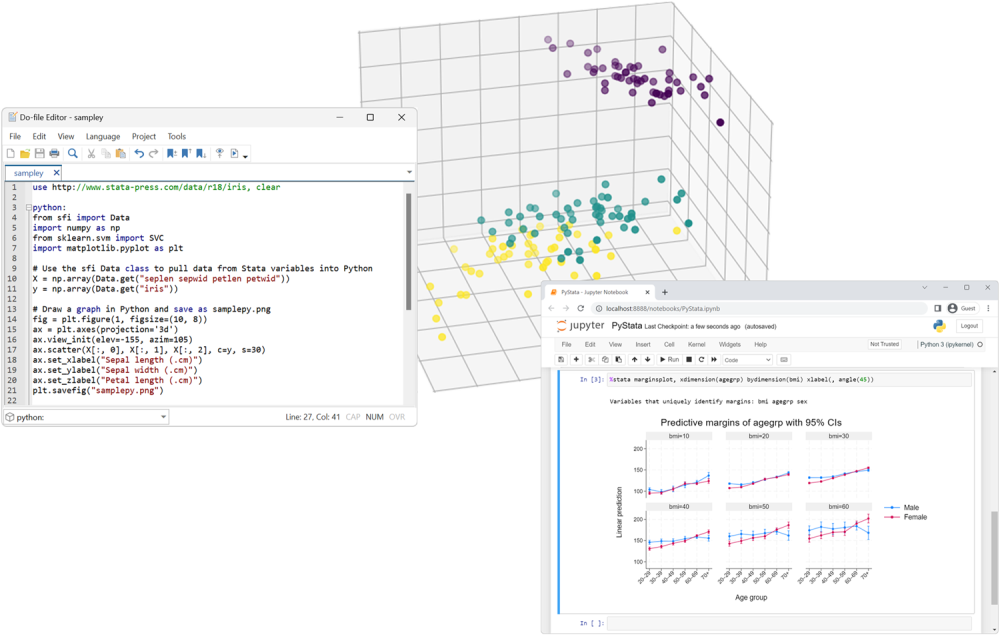
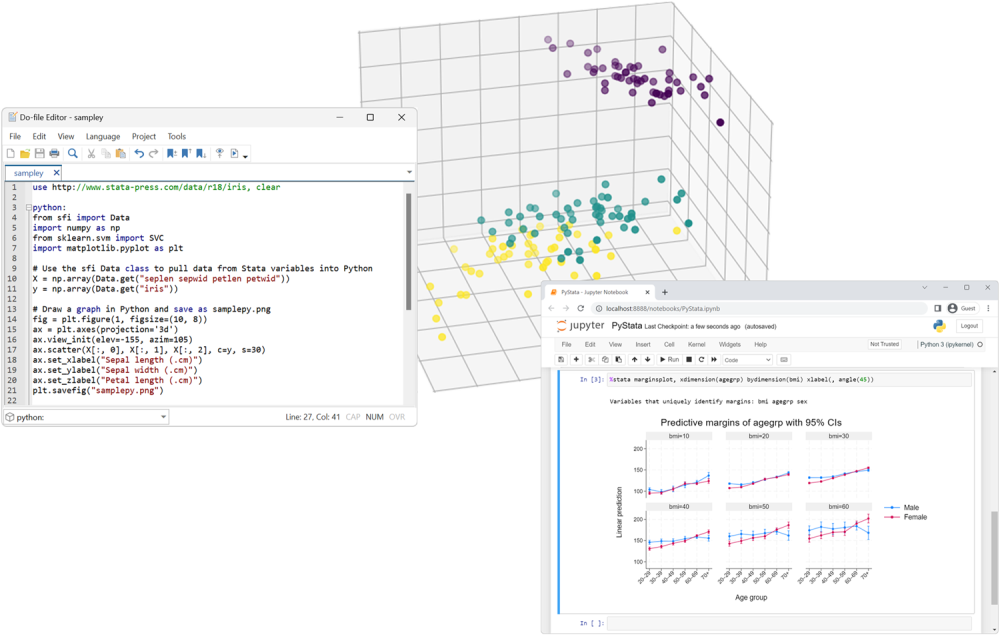
Des recherches véritablement reproductibles
On parle beaucoup de recherche reproductible. Stata s'y consacre depuis plus de 30 ans.
Nous ajoutons constamment de nouvelles fonctionnalités ; nous avons même fondamentalement modifié des éléments de langage. Peu importe. Stata est le seul logiciel statistique intégrant le versioning. Si vous avez écrit un script pour effectuer une analyse en 1985, ce même script fonctionnera toujours et produira les mêmes résultats aujourd'hui. Vous pouvez lire tous les jeux de données créés en 1985 aujourd'hui. Et il en sera de même en 2050. Stata pourra exécuter tout ce que vous faites aujourd'hui.
Nous prenons la reproductibilité au sérieux.
Documentation réelle
Lorsqu'il s'agit d'effectuer vos analyses ou de comprendre les méthodes que vous utilisez, Stata ne vous laisse pas tomber et ne vous oblige pas à commander des livres pour apprendre chaque détail.
Chacune de nos fonctionnalités de gestion de données est entièrement expliquée, documentée et illustrée par des exemples concrets. Chaque estimateur est entièrement documenté et inclut plusieurs exemples basés sur des données réelles, accompagnés de discussions concrètes sur l'interprétation des résultats. Ces exemples vous fournissent les données nécessaires pour travailler avec Stata et même étendre vos analyses. Nous vous proposons un guide de démarrage rapide pour chaque fonctionnalité, présentant certaines des utilisations les plus courantes. Besoin de plus de détails ? Nos sections Méthodes et formules détaillent les calculs effectués, et nos références vous orientent vers des informations complémentaires.
Stata est un logiciel volumineux, avec une documentation abondante : plus de 18 000 pages réparties en 35 manuels. Pas d'inquiétude : saisissez « Aide mon sujet » et Stata effectuera une recherche parmi ses mots-clés, ses index et même les logiciels proposés par la communauté pour vous fournir tout ce dont vous avez besoin sur votre sujet. Tout est disponible directement dans Stata.
De confiance
Nous ne nous contentons pas de programmer des méthodes statistiques, nous les validons.
Les résultats obtenus avec un estimateur Stata reposent sur des comparaisons avec d'autres estimateurs, des simulations de Monte-Carlo de cohérence et de couverture, et des tests approfondis effectués par nos statisticiens. Chaque Stata que nous livrons a passé avec succès une suite de certification comprenant 4,1 millions de lignes de code de test produisant 5,8 millions de lignes de sortie. Nous certifions chaque chiffre et chaque extrait de texte de ces 5,8 millions de lignes de sortie.
Fiable
Depuis plus de 35 ans, StataCorp est fidèle à ses utilisateurs en enrichissant le logiciel Stata de nouvelles méthodes statistiques et des technologies de pointe en matière de reporting, de visualisation et de manipulation de données, ainsi que d'interface utilisateur. Forts de notre longue expérience en matière de versions, nous nous engageons à fournir en permanence des logiciels stables et fiables à notre communauté diversifiée de chercheurs et de praticiens.
Mise à jour continue
Rester sur la version la plus récente de Stata est désormais plus facile que jamais.
StataCorp développe continuellement de nouvelles fonctionnalités pour améliorer le logiciel Stata, des méthodes statistiques les plus récentes aux meilleurs outils de reporting, de visualisation de données et d'interface utilisateur. Avec StataNow™, de nouvelles fonctionnalités sont déployées tout au long de la version actuelle jusqu'à la prochaine version majeure. Ces fonctionnalités sont priorisées dans le cycle de développement afin d'être disponibles dès leur disponibilité et d'être immédiatement utilisables par les utilisateurs.
Facile à utiliser
Rester sur la version la plus récente de Stata est désormais plus facile que jamais.
Toutes les fonctionnalités de Stata sont accessibles via des menus, des boîtes de dialogue, des panneaux de configuration, un éditeur de données, un gestionnaire de variables, un éditeur de graphiques et même un générateur de diagrammes SEM. Vous pouvez naviguer dans n'importe quelle analyse en un clic.
Si vous ne souhaitez pas écrire de commandes et de scripts, vous n'êtes pas obligé de le faire.
Même en pointant et en cliquant, vous pouvez enregistrer tous vos résultats et les inclure ultérieurement dans des rapports. Vous pouvez même sauvegarder les commandes créées par vos actions et reproduire ultérieurement votre analyse complète.
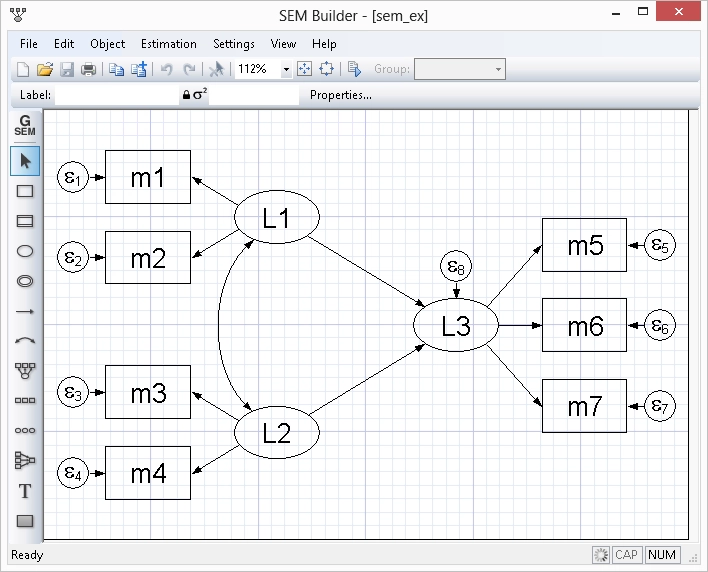
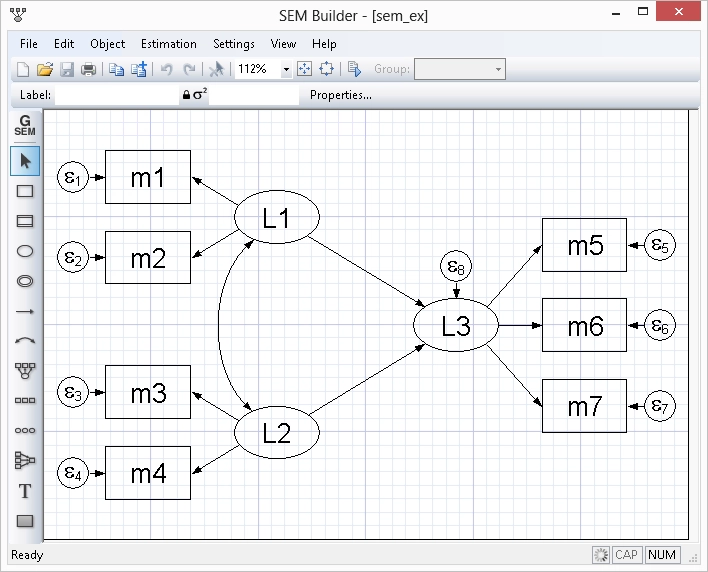
Facile à cultiver avec
Les commandes Stata pour l'exécution des tâches sont intuitives et faciles à prendre en main. Mieux encore, tout ce que vous apprenez sur l'exécution d'une tâche peut être appliqué à d'autres tâches. Par exemple, ajoutez simplement « if gender=="female" » à n'importe quelle commande pour limiter votre analyse aux femmes de votre échantillon. Ajoutez simplement « vce(robust) » à n'importe quel estimateur pour obtenir des erreurs types et des tests d'hypothèses robustes à de nombreuses hypothèses courantes.
La cohérence est encore plus poussée. Ce que vous apprenez sur les commandes de gestion des données s'applique souvent aux commandes d'estimation, et inversement. Il existe également une suite complète de commandes de post-estimation permettant d'effectuer des tests d'hypothèse, de former des combinaisons linéaires et non linéaires, de faire des prédictions, de former des contrastes et même d'effectuer des analyses marginales avec des graphiques d'interaction. Ces commandes fonctionnent de la même manière après pratiquement tous les estimateurs.
Le séquençage des commandes pour lire et nettoyer les données, puis effectuer des tests statistiques et des estimations, et enfin communiquer les résultats, est au cœur d'une recherche reproductible. Stata rend ce processus accessible à tous les chercheurs.
Facile à automatiser
Tout le monde a des tâches à effectuer en permanence : créer un type particulier de variable, produire une table particulière, exécuter une séquence d'étapes statistiques, calculer une RMSE, etc. Les possibilités sont infinies. Stata propose des milliers de procédures intégrées, mais certaines de vos tâches sont relativement uniques ou nécessitent une exécution spécifique.
Si vous avez écrit un script pour effectuer votre tâche sur un ensemble de données donné, il est facile de transformer ce script en quelque chose qui peut être utilisé sur tous vos ensembles de données, sur n'importe quel ensemble de variables et sur n'importe quel ensemble d'observations.
Facile à étendre
Certaines des choses que vous automatisez peuvent être si utiles que vous souhaitez les partager avec vos collègues, voire les rendre accessibles à tous les utilisateurs de Stata. C'est très simple. Avec un peu de code, vous pouvez transformer un script d'automatisation en commande Stata. Une commande prenant en charge les fonctionnalités standard des commandes officielles de Stata. Une commande utilisable de la même manière que les commandes officielles.
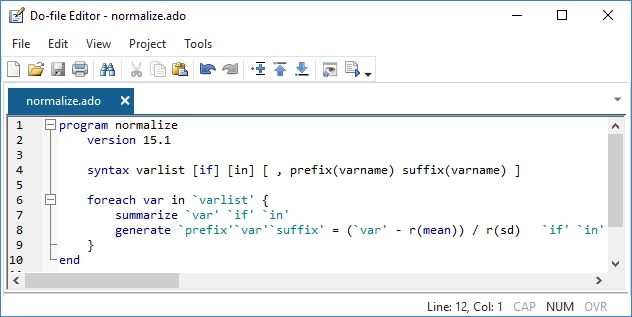
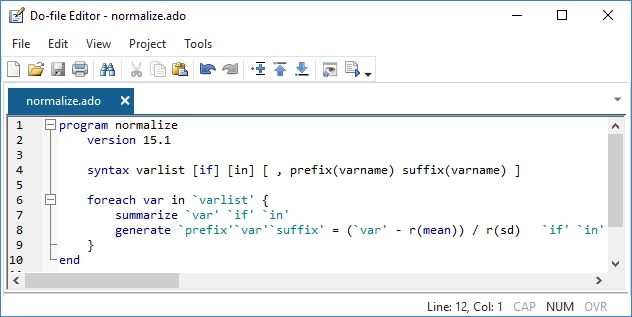
Programmation avancée
Stata inclut également un langage de programmation avancé : Mata.
Mata possède les structures, les pointeurs et les classes que vous attendez dans votre langage de programmation et ajoute un support direct pour la programmation matricielle.
Bien que Stata ne nécessite pas de savoir programmer, il est rassurant de savoir qu'un langage de programmation rapide et complet fait partie intégrante de Stata. Mata est à la fois un environnement interactif pour la manipulation de matrices et un environnement de développement complet capable de produire du code compilé et optimisé. Il inclut des fonctionnalités spécifiques pour le traitement des données de panel, effectue des opérations sur des matrices réelles ou complexes, offre une prise en charge complète de la programmation orientée objet et est entièrement intégré à tous les aspects de Stata. Stata offre également une intégration Python complète, vous permettant d'exploiter toute la puissance de Python directement depuis votre code Stata.
Stata dispose également de PyStata, qui fournit une intégration Python complète, vous permettant d'exploiter toute la puissance de Python directement à partir de votre code Stata et d'exploiter toute la puissance de Stata à partir de votre code Python.
Stata vous permet même d'intégrer des plugins C, C++ et Java à vos programmes Stata via une API native pour chaque langage. Vous pouvez même intégrer du code Java directement dans votre code Stata !
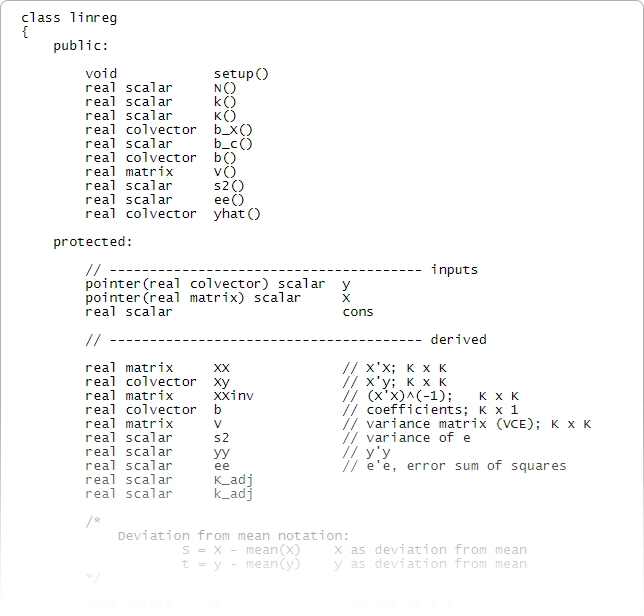
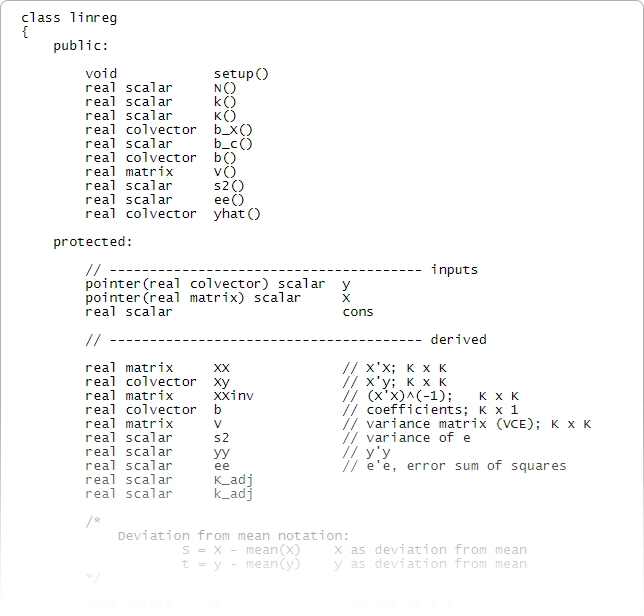
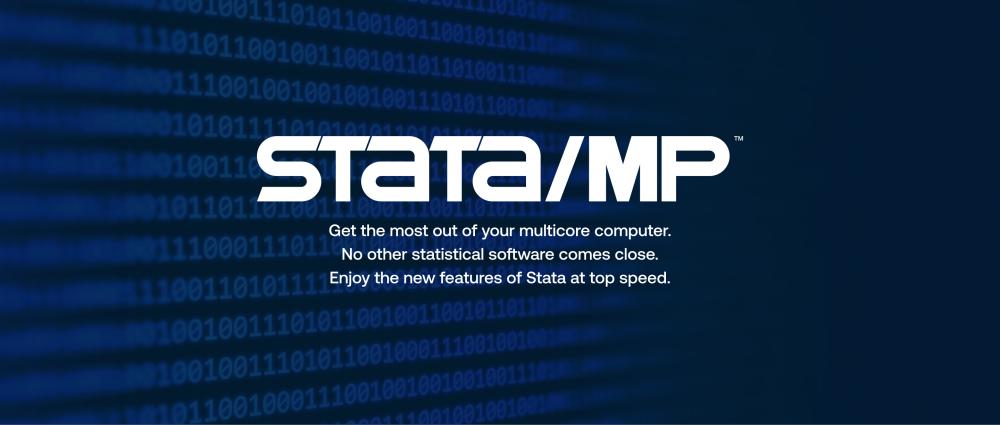
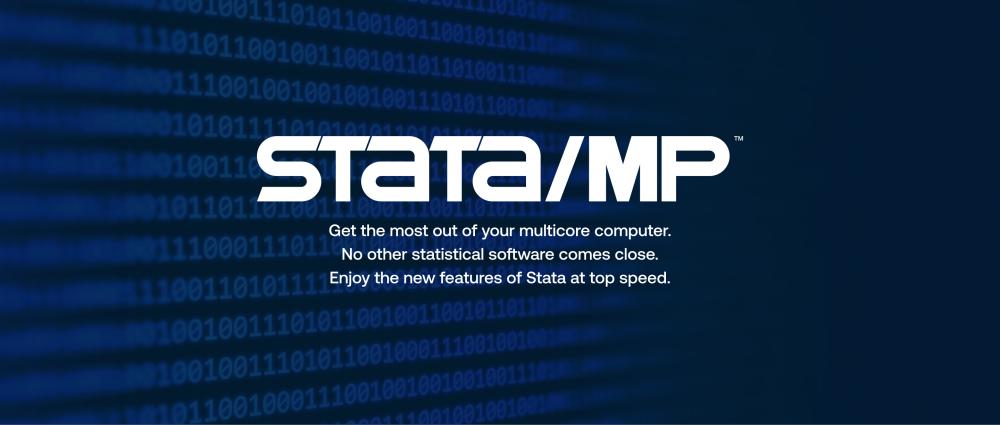
Fonctionnalités apportées par la communauté
Stata est tellement programmable que les développeurs et les utilisateurs ajoutent chaque jour de nouvelles fonctionnalités pour répondre aux demandes croissantes des chercheurs d'aujourd'hui.
Grâce aux capacités Internet de Stata, de nouvelles fonctionnalités et des mises à jour officielles peuvent être installées sur Internet en un seul clic.
Support technique de classe mondiale
Tous les utilisateurs enregistrés de la version actuelle de Stata (Stata 18) bénéficient d'une assistance technique gratuite. Si vous n'avez pas enregistré votre exemplaire de Stata, veuillez remplir le formulaire d'inscription en ligne.
Notre équipe dédiée de programmeurs et de statisticiens experts Stata est là pour répondre à vos questions techniques. Des solutions complexes de gestion de données à l'obtention d'un graphique parfait, en passant par l'explication d'une erreur standard robuste et la spécification de votre modèle multiniveau, nous avons les réponses.
Compatible multiplateforme
Stata fonctionne sur Windows, Mac et Linux/Unix ; cependant, nos licences ne sont pas spécifiques à chaque plateforme. Ainsi, si vous possédez un ordinateur portable Mac et un ordinateur de bureau Windows, vous n'avez pas besoin de deux licences distinctes pour utiliser Stata. Vous pouvez installer votre licence Stata sur n'importe quelle plateforme prise en charge. Les jeux de données, programmes et autres données Stata peuvent être partagés entre différentes plateformes sans conversion. Vous pouvez également importer rapidement et facilement des jeux de données depuis d'autres logiciels statistiques, feuilles de calcul et bases de données.
Largement utilisé
Utilisé par les chercheurs depuis plus de 35 ans, Stata fournit tout ce dont vous avez besoin pour la science des données : manipulation de données, visualisation, statistiques et rapports automatisés.
Sélectionnez votre discipline et voyez comment Stata peut travailler pour vous.
Features For Data Scientists
Data wrangling
Scrape data from the web, import it from standard formats, or pull it in via SQL with JDBC or ODBC. Match-merge, link, append, reshape, transpose, sort, filter. Stata handles Unicode, frames (multiple datasets in memory), BLOBs, regular expressions, and more, whether working with hundreds of thousands or even billions of data points.
Automated reporting and customizable tables
Use Markdown to create Word documents and HTML files with embedded Stata code, output, and graphs. Automate Word, PDF, or Excel reports with both high-level export capabilities and low-level fine-grained programmatic access to automate production of the documents your team needs. Customize tables to clearly communicate results, and export your tables to Word, PDF, HTML, LaTeX, Excel, or Markdown.
Visualisation
Create graphs and customize them programmatically or interactively with the Graph Editor. Edits can even be recorded and "replayed" on other graphs for reproducibility. Export to industry standard formats suitable for web (SVG, PNG) or print (PDF, TIFF, EPS, PS).
Programming
Automate your entire workflow with both scripts and full-blown programming features like classes, structures, and pointers. A unique feature of Stata's programming environment is Mata, a fast and compiled matrix programming language. Of course, it has all the advanced matrix operations you need. It also has access to the power of LAPACK. What's more, it has built-in solvers and optimizers to make implementing your own estimator easier. And you can leverage all of Stata's estimation features and other features from within Mata.
PyStata—Python integration
Interact Stata code with Python code. You can seamlessly pass data and results between Stata and Python. You can use Stata within Jupyter Notebook and other IPython environments. You can call Python libraries such as NumPy, matplotlib, Scrapy, scikit-learn, and more from Stata. You can use Stata analyses from within Python.
Interoperability
Connect to external code via Python, Java, and C++ plugins. Write Python or Java code directly within your Stata code. Control Stata via Jupyter Notebook, OLE Automation, or call it in batch mode. Write custom SQL statements with JDBC and ODBC to extract from or populate databases. Access H2O clusters.
Statistics and modeling
Incorporate state-of-the-art statistical models and results in your workflow. Find groups in your data using unsupervised techniques including cluster analysis, principal components, factor analysis, multidimensional scaling, and correspondence analysis. Understand your groups even better using latent class analysis. When your analysis calls for supervised techniques, Stata has flexible nonparametric methods and an array of regression models from linear and logistic models to mixture models. Stata keeps up when your data call for special techniques. You have access to methods that understand and take advantage of the structure in time series, panel data, survival data, complex survey data, spatial data, and multilevel data. Stata provides the most approachable implementations of Bayesian methods and structural equation modeling available anywhere. You can request bootstrap methods for virtually any estimator. When your analysis calls for it, Stata automates other replication methods and simulations.
Reproducibility
Stata is the only software for data science and statistical analysis featuring a comprehensive version control system that ensures your code continues to run, unaltered, even after updates or new versions are released. No need to keep around multiple legacy installations to avoid breaking your system; Stata code from 25 years ago can still be run without modification. Datasets, graphs, scripts, programs, and more are 100% cross-platform and backward compatible.
Lasso
Use lasso and elastic net for model selection and prediction. And when you want to estimate effects and test coefficients for a few variables of interest, inferential methods provide estimates for these variables while using lassos to select from among a potentially large number of control variables. You can even account for endogenous covariates. Whether your goal is model selection, prediction, or inference, you can use Stata's lasso features with your continuous, binary, count, or time-to-event outcomes.
Features for Economists
Panel data
Take full advantage of the extra information that panel data provide while simultaneously handling the peculiarities of panel data. Study the time-invariant features within each panel, the relationships across panels, and how outcomes of interest change over time. Fit linear models or nonlinear models for binary, count, ordinal, censored, or survival outcomes with fixed-effects, random-effects, or population-averaged estimators. Fit dynamic models or models with endogeneity.
Time series
Handle the statistical challenges inherent to time-series data—autocorrelations, common factors, autoregressive conditional heteroskedasticity, unit roots, cointegration, and much more. Analyze univariate time series using ARIMA, ARFIMA, Markov-switching models, ARCH and GARCH models, and unobserved-components models. Compare ARIMA or ARFIMA models using AIC, BIC, and HQIC, and select the best number of autoregressive and moving-average terms. Analyze multivariate time series using VAR, structural VAR, VEC, multivariate GARCH, dynamic-factor models, and state-space models. Compute and graph impulse responses. Test for unit roots. Perform Bayesian time-series analysis.
Cross-sectional models
Fit classical linear models of the relationship between a continuous outcome, such as wage, and the determinants of wage, such as education level, age, experience, and economic sector. If your response is binary (for example, employed or unemployed), ordinal (education level), count (number of children), or censored (ticket sales in an existing venue), don't worry. Stata has maximum likelihood estimators—probit, ordered probit, Poisson, tobit, and many others—that estimate the relationship between such outcomes and their determinants. A vast array of tools is available to analyze such models. Predict outcomes and their confidence intervals. Test equality of parameters, or any linear or nonlinear combination of parameters.
Endogeneity and selection
When explanatory variables are related to omitted observable variables, or when they are related to unobservable variables, or when there is selection bias, then causal relationships are confounded and parameter estimates from standard estimators produce inconsistent estimates of the true relationships. Stata can fit consistent models when there is such endogeneity or selection—whether your outcome variable is continuous, binary, count, or ordinal and whether your data are cross-sectional or panel. Stata can even combine endogenous covariates, selection, and treatment effects in the same model.
Causal inference/Treatment effects
Estimate experimental-style causal effects from observational data; for instance, estimate the effect of a job training program on employment or the effect of a subsidy on production. Fit models for continuous, binary, count, fractional, and survival outcomes with binary or multivalued treatments using inverse-probability weighting (IPW), propensity-score matching, nearest-neighbor matching, regression adjustment, or doubly robust estimators. Fit models with exogenous or endogenous treatments. After estimation, test the overlap assumption and covariate balance. Add endogenous covariates and sample selection to some treatment-effects estimators. In the presence of group and time effects, you can use difference-in-differences (DID) and triple-differences (DDD) estimators. In the presence of high-dimensional covariates, you can use lasso. If causal effects are mediated through another variable, use causal mediation with mediate to disentangle direct and indirect effects.
Marginal effects and marginal means
Marginal effects and marginal means let you analyze and visualize the relationships between your outcome variable and your covariates, even when that outcome is binary, count, ordinal, categorical, or censored (tobit). Estimate population-averaged marginal effects or evaluate marginal effects at interesting or representative values of the covariates. Analyze the effect of interactions. You can even trace out the marginal effect over a range of interesting covariate values or covariate interactions. You can do all of this with marginal means (sometimes called potential-outcome means), even when your “mean” is a probability of a positive outcome or a count from a Poisson model. If you have panel data and random effects, these effects are automatically integrated out to provide marginal (that is, population-averaged) effects.
Choice models
Model your discrete choice data. If your outcome is, for instance, a choice to travel by bus, train, car, or airplane, you can fit a conditional logit, multinomial probit, or mixed logit model. Is your outcome instead a ranking of prefered travel methods? Fit a rank-ordered probit or rank-ordered logit model. Regardless of the model fit, you can use the margins to easily interpret the results. Estimate how much wait times at the airport affect the probability of traveling by air or even by train.
GMM
GMM (generalized method of moments) can be used to fit almost any statistical model, including both exactly identified and overidentified estimation problems. Overidentified problems arise when you have endogeneity, correlation in dynamic panels, sample selection, and many other situations. With Stata, you estimate these models by simply writing your moments and enclosing the parameters in curly braces. You can easily fit cross-sectional, time-series, panel-data, or survival-data models and test your overidentifying restrictions.
Demand systems
Fit demand systems to explore consumers' demand for goods and services. Given a budget and a bundle of goods and services, determine the expenditure and price elasticities for these goods. Choose between the Cobb–Douglas system, Stone's linear expenditure system, the translog indirect utility demand system, the almost ideal demand system (AIDS), the quadratic almost ideal demand system (QUAIDS), and others.
Lasso
Use lasso and elastic net for model selection and prediction. And when you want to estimate effects and test coefficients for a few variables of interest, inferential methods provide estimates for these variables while using lassos to select from among a potentially large number of control variables. You can even account for endogenous covariates. Whether your goal is model selection, prediction, or inference, you can use Stata's lasso features with your continuous, binary, count, or time-to-event outcomes.
Programming
Want to program your own commands to perform estimation, perform data management, or implement other new features? Stata is programmable, and thousands of Stata users have implemented and published thousands of community-contributed commands. These commands look and act just like official Stata commands and are easily installed for free over the Internet from within Stata. A unique feature of Stata's programming environment is Mata, a fast and compiled language with support for matrix types. Of course, it has all the advanced matrix operations you need. It also has access to the power of LAPACK. What's more, it has built-in solvers and optimizers to make implementing your own maximum likelihood, GMM, or other estimators easier. And you can leverage all of Stata's estimation and other features from within Mata. Many of Stata's official commands are themselves implemented in Mata.
PyStata - Python integration
Interact Stata code with Python code. You can seamlessly pass data and results between Stata and Python. You can use Stata within Jupyter Notebook and other IPython environments. You can call Python libraries such as NumPy, matplotlib, Scrapy, scikit-learn, and more from Stata. You can use Stata analyses from within Python.
Forecasting
Build multiequation models, and produce forecasts of levels, trends, rates, etc. Whether you have a small model with a few equations or a complete model of the economy with thousands of equations, Stata can help you build that model and produce forecasts. Your model can include both estimated relationships and known identities. You can easily create and compare forecasts under different scenarios, create static and dynamic forecasts, and even estimate stochastic confidence intervals. You can create your model by using an intuitive command syntax or by using the interactive forecasting control panel.
Survival Analysis
Analyze duration outcomes—outcomes measuring the time to an event such as failure or death—using Stata's specialized tools for survival analysis. Account for the complications inherent in survival data, such as sometimes not observing the event (right-, left-, and interval-censoring), individuals entering the study at differing times (delayed entry), and individuals who are not continuously observed throughout the study (gaps). You can estimate and plot the probability of survival over time. Or model survival as a function of covariates using Cox, Weibull, lognormal, and other regression models. Predict hazard ratios, mean survival time, and survival probabilities. Do you have groups of individuals in your study? Adjust for within-group correlation with a random-effects or shared-frailty model. If you have many potential covariates, use lasso cox and elasticnet cox for model selection and prediction.
Bayesian analysis
Perform Bayesian econometrics analysis using one of the Markov chain Monte Carlo (MCMC) methods. You can choose from various supported models, such as panel-data, hierarchical, VAR, and DSGE models, or you can even program your own. Extensive tools are available to check convergence, including multiple chains. Compute posterior mean estimates and credible intervals for model parameters and functions of model parameters. You can perform both interval- and model-based hypothesis testing. Compare models using Bayes factors. Compute model fit using posterior predictive values. Generate predictions and forecasts. If you want to account for model uncertainty in your regression model, use Bayesian model averaging.
Survey methods
Whether your data require a simple weighted adjustment because of differential sampling rates or you have data from a complex multistage survey, Stata's survey features can provide you with correct standard errors and confidence intervals for your inferences. Simply specify the relevant characteristics of your sampling design, such as sampling weights (including weights at multiple stages), clustering (at one, two, or more stages), stratification, and poststratification. After that, most of Stata's estimation commands can adjust their estimates to correct for your sampling design.
Meta-analysis
Combine results of multiple studies to estimate an overall effect. Use forest plots to visualize results. Use subgroup analysis and meta-regression to explore study heterogeneity. Use funnel plots and formal tests to explore publication bias and small-study effects. Use trim-and-fill analysis to assess the impact of publication bias on results. Perform cumulative and leave-one-out meta-analysis. Perform univariate, multilevel, and multivariate meta-analysis. Use the meta suite, or let the Control Panel interface guide you through your entire meta-analysis.
Automated reporting and customizable tables
Stata is designed for reproducible research, including the ability to create dynamic documents incorporating your analysis results. Create Word or PDF files, populate Excel worksheets with results and format them to your liking, and mix Markdown, HTML, Stata results, and Stata graphs, all from within Stata. Create tables that compare regression results or summary statistics, use default styles or apply your own, and export your tables to Word, PDF, HTML, LaTeX, Excel, or Markdown and include them in your reports.
Features for Education
Multilevel mixed-effects models
Whether the groupings in your data arise in a nested fashion (students nested in classrooms and classrooms nested in schools) or in a nonnested fashion (elementary school crossed with middle school), you can fit a multilevel model to account for the lack of independence within these groups. Fit models for continuous, binary, count, ordinal, and survival outcomes. Estimate variances of random intercepts and random coefficients. Compute intraclass correlations. Predict random effects. Estimate relationships that are population averaged over the random effects.
Structural equation modeling (SEM)
Estimate mediation effects, analyze the relationship between an unobserved latent concept such as verbal abilities and the observed variables that measure verbal abilities, or fit a model with complex relationships among both latent and observed variables. Fit models with continuous, binary, count, and ordinal outcomes. Even fit hierarchical models with groups of correlated observations such as children within the same schools. Evaluate model fit. Compute indirect and total effects. Fit models by drawing a path diagram or using the straightforward command syntax.
General Linear Models
Fit one- and two-way models. Or fit models with three, four, or even more factors. Analyze data with nested factors, with fixed and random factors, or with repeated measures. Use ANCOVA models when you have continuous covariates and MANOVA models when you have multiple outcome variables. Further explore the relationships between your outcome and predictors by estimating effect sizes and computing least-squares and marginal means. Perform contrasts and pairwise comparisons. Analyze and plot interactions.
IRT (item response theory)
Explore the relationship between unobserved latent characteristics such as mathematical aptitude and the probability of correctly answering test questions (items). Or explore the relationship between teacher job satisfaction and self-reported responses to questions related to job statisfaction. IRT can be used to create measures of such unobserved traits or place individuals on a scale measuring the trait. It can also be used to select the best items for measuring a latent trait. IRT models are available for binary, graded, rated, partial-credit, and nominal response items. Visualize the relationships using item characteristic curves, and measure overall test performance using test information functions.
Linear, binary and count regressions
Fit classical linear regression models of the relationship between a continuous outcome, such as a reading test score, and the determinants of the score, such as teaching method and the student's reading level in the previous grade. If your response is binary (for example, pass or fail test), ordinal (education level), count (number of students), or categorical (private, public, or home school), don't worry. Stata has maximum likelihood estimators—logistic, ordered logistic, Poisson, multinomial logit, and many others—that estimate the relationship between such outcomes and their determinants. A vast array of tools is available after fitting such models. Predict outcomes and their confidence intervals. Test equality of parameters. Compute linear and nonlinear combinations of parameters.
Linear, binary and count regressions
Account for missing data in your sample using multiple imputation. Choose from univariate and multivariate methods to impute missing values in continuous, censored, truncated, binary, ordinal, categorical, and count variables. Then, in a single step, estimate parameters using the imputed datasets, and combine results. Fit a linear model, logit model, Poisson model, hierarchical model, survival model, or one of the many other supported models. Use the mi command, or let the Control Panel interface guide you through your entire MI analysis.
Choice models
Model your discrete choice data. If your outcome is, for instance, a choice to travel by bus, train, car, or airplane, you can fit a conditional logit, multinomial probit, or mixed logit model. Is your outcome instead a ranking of prefered travel methods? Fit a rank-ordered probit or rank-ordered logit model. Regardless of the model fit, you can use the margins to easily interpret the results. Estimate how much wait times at the airport affect the probability of traveling by air or even by train.
Contrasts, marginal means and profile plots
Quickly and easily obtain contrasts for categorical variables and their interactions. R.edlevel will give you all the contrasts of education level with a reference category. A.edlevel will give you each paired contrast with the next higher education level. There are many more named contrasts, and you can specify your own. If you don't like typing, use a dialog box to select your contrasts. Marginal means are just a simple command or mouse click away after almost any estimation command. Evaluating interaction effects, the effects of moderating variables, is just as easy. And this is not just for linear models, but for models with binary, ordinal, and count outcomes. Even for hierarchical models with correct handling of random effects. A simple command or a few mouse clicks will get you a profile plot of any of these results.
Power, precision and sample size
Before you conduct your experiment, determine the sample size needed to detect meaningful effects without wasting resources. Do you intend to compute CIs for means or variances or perform tests for proportions or correlations? Do you plan to fit a Cox proportional hazards model or compare survivor functions using a log-rank test? Do you want to use a Cochran—Mantel—Haenszel test of association or a Cochran—Armitage trend test? Use Stata's power command to compute power and sample size, create customized tables, and automatically graph the relationships between power, sample size, and effect size for your planned study. Or use the ciwidth command to do the same but for CIs instead of hypothesis tests by computing the required sample size for the desired CI precision. Or use gsdesign to compute stopping boundaries and the required sample sizes for group sequential designs. Instead of commands, use the interactive Control Panel to perform your analysis.
Causal Inference
Estimate experimental-style causal effects from observational data. With Stata's treatment-effects estimators, you can use a potential-outcomes (counterfactuals) framework to estimate, for instance, the effect of family structure on child development or the effect of unemployment on anxiety. Fit models for continuous, binary, count, fractional, and survival outcomes with binary or multivalued treatments using inverse-probability weighting (IPW), propensity-score matching, nearest-neighbor matching, regression adjustment, or doubly robust estimators. If the assignment to a treatment is not independent of the outcome, you can use an endogenous treatment-effects estimator. In the presence of group and time effects, you can use difference-in-differences (DID) and triple-differences (DDD) estimators. In the presence of high-dimensional covariates, you can use lasso. If causal effects are mediated through another variable, use causal mediation with mediate to disentangle direct and indirect effects.
Multivariate methods
Use multivariate analyses to evaluate relationships among variables from many different perspectives. Perform multivariate tests of means, or fit multivariate regression and MANOVA models. Explore relationships between two sets of variables, such as aptitude measurements and achievement measurements, using canonical correlation. Examine the number and structure of latent concepts underlying a set of variables using exploratory factor analysis. Or use principal component analysis to find underlying structure or to reduce the number of variables used in a subsequent analysis. Discover groupings of observations in your data using cluster analysis. If you have known groups in your data, describe differences between them using discriminant analysis.
Automated reporting and customizable tables
Stata is designed for reproducible research, including the ability to create dynamic documents incorporating your analysis results. Create Word or PDF files, populate Excel worksheets with results and format them to your liking, and mix Markdown, HTML, Stata results, and Stata graphs, all from within Stata. Create tables that compare regression results or summary statistics, use default styles or apply your own, and export your tables to Word, PDF, HTML, LaTeX, Excel, or Markdown and include them in your reports.
Bayesian analysis
Perform Bayesian econometrics analysis using one of the Markov chain Monte Carlo (MCMC) methods. You can choose from various supported models, such as panel-data, hierarchical, VAR, and DSGE models, or you can even program your own. Extensive tools are available to check convergence, including multiple chains. Compute posterior mean estimates and credible intervals for model parameters and functions of model parameters. You can perform both interval- and model-based hypothesis testing. Compare models using Bayes factors. Compute model fit using posterior predictive values. Generate predictions and forecasts. If you want to account for model uncertainty in your regression model, use Bayesian model averaging.
Meta-analysis
Combine results of multiple studies to estimate an overall effect. Use forest plots to visualize results. Use subgroup analysis and meta-regression to explore study heterogeneity. Use funnel plots and formal tests to explore publication bias and small-study effects. Use trim-and-fill analysis to assess the impact of publication bias on results. Perform cumulative and leave-one-out meta-analysis. Perform univariate, multilevel, and multivariate meta-analysis. Use the meta suite, or let the Control Panel interface guide you through your entire meta-analysis.
Jupyter Notebook with Stata
Jupyter Notebook is widely used by researchers and scientists to share their ideas and results for collaboration and innovation. It is an easy-to-use web application that allows you to combine code, visualizations, mathematical formulas, narrative text, and other rich media in a single document (a "notebook") for interactive computing and developing. You can invoke Stata and Mata from Jupyter Notebook with the IPython (interactive Python) kernel. This means you can combine the capabilities of both Python and Stata in a single environment to make your work easily reproducible and shareable with others.
Features for Epidemiologists
Epidemiological tables
Want to analyze data from a prospectiv321 laddence") study, cohort study, case–control study, or matched case–control study? Stata's tables for epidemiologists make it easy to summarize your data and compute statistics such as incidence-rate ratios, incidence-rate differences, risk ratios, risk differences, odds ratios, and attributable fractions. You can analyze stratified data too—compute Mantel–Haenszel combined estimates, perform tests of homogeneity, and standardize estimates. If you have an ordinal rather than binary exposure, you can perform a test for a trend.
Survival analysis
Analyze duration outcomes—outcomes measuring the time to an event such as failure or death—using Stata's specialized tools for survival analysis. Account for the complications inherent in survival data, such as sometimes not observing the event (right-, left-, and interval-censoring), individuals entering the study at differing times (delayed entry), and individuals who are not continuously observed throughout the study (gaps). You can estimate and plot the probability of survival over time. Or model survival as a function of covariates using Cox, Weibull, lognormal, and other regression models. Predict hazard ratios, mean survival time, and survival probabilities. Do you have groups of individuals in your study? Adjust for within-group correlation with a random-effects or shared-frailty model. If you have many potential covariates, use lasso cox and elasticnet cox for model selection and prediction.
Linear, binary and count regressions
Fit classical ANOVA and linear regression models of the relationship between a continuous outcome, such as weight, and the determinants of weight, such as height, diet, and level of exercise. If your response is binary, ordinal, categorical, or count, don't worry. Stata has estimators for these types of outcomes too. Use logistic regression to adjust odds ratios for confounding variables. Estimate incidence rates using a Poisson model. Analyze matched case–control data with conditional logistic regression. A vast array of tools is available after fitting such models. Predict outcomes and their confidence intervals. Test equality of parameters. Compute linear and nonlinear combinations of parameters.
Survey methods
Whether your data require a simple weighted adjustment because of differential sampling rates or you have data from a complex multistage survey, Stata's survey features can provide you with correct standard errors and confidence intervals for your inferences. Simply specify the relevant characteristics of your sampling design, such as sampling weights (including weights at multiple stages), clustering (at one, two, or more stages), stratification, and poststratification. After that, most of Stata's estimation commands can adjust their estimates to correct for your sampling design.
Marginal means, contrasts and interactions
Marginal means and contrasts let you analyze the relationships between your outcome variable and your predictors, even when your outcome is binary, count, ordinal, or categorical. For instance, after you fit a logistic regression of a disease on an exposure variable and other covariates, your marginal means may be population-averaged risks. Or you can set the covariates to interesting values to compute adjusted risks and then use contrasts to get adjusted risk differences. After fitting almost any model in Stata, you can analyze the effect of covariate interactions and easily create plots to visualize those interactions.
Power, precision and sample size
Before you conduct your experiment, determine the sample size needed to detect meaningful effects without wasting resources. Do you intend to compute CIs for means or variances or perform tests for proportions or correlations? Do you plan to fit a Cox proportional hazards model or compare survivor functions using a log-rank test? Do you want to use a Cochran—Mantel—Haenszel test of association or a Cochran—Armitage trend test? Use Stata's power command to compute power and sample size, create customized tables, and automatically graph the relationships between power, sample size, and effect size for your planned study. Or use the ciwidth command to do the same but for CIs instead of hypothesis tests by computing the required sample size for the desired CI precision. Or use gsdesign to compute stopping boundaries and the required sample sizes for group sequential designs. Instead of commands, use the interactive Control Panel to perform your analysis.
Meta-analysis
Combine results of multiple studies to estimate an overall effect. Use forest plots to visualize results. Use subgroup analysis and meta-regression to explore study heterogeneity. Use funnel plots and formal tests to explore publication bias and small-study effects. Use trim-and-fill analysis to assess the impact of publication bias on results. Perform cumulative and leave-one-out meta-analysis. Perform univariate, multilevel, and multivariate meta-analysis. Use the meta suite, or let the Control Panel interface guide you through your entire meta-analysis.
Causal inference
Estimate experimental-style causal effects from observational data. With Stata's treatment-effects estimators, you can use a potential-outcomes (counterfactuals) framework to estimate, for instance, the effect of family structure on child development or the effect of unemployment on anxiety. Fit models for continuous, binary, count, fractional, and survival outcomes with binary or multivalued treatments using inverse-probability weighting (IPW), propensity-score matching, nearest-neighbor matching, regression adjustment, or doubly robust estimators. If the assignment to a treatment is not independent of the outcome, you can use an endogenous treatment-effects estimator. In the presence of group and time effects, you can use difference-in-differences (DID) and triple-differences (DDD) estimators. In the presence of high-dimensional covariates, you can use lasso. If causal effects are mediated through another variable, use causal mediation with mediate to disentangle direct and indirect effects.
Multiple imputation
Account for missing data in your sample using multiple imputation. Choose from univariate and multivariate methods to impute missing values in continuous, censored, truncated, binary, ordinal, categorical, and count variables. Then, in a single step, estimate parameters using the imputed datasets, and combine results. Fit a linear model, logit model, Poisson model, multilevel model, survival model, or one of the many other supported models. Use the mi command, or let the Control Panel interface guide you through your entire MI analysis.
Multilevel mixed-effects models
Whether the groupings in your data arise in a nested fashion (patients nested in clinics and clinics nested in regions) or in a nonnested fashion (regions crossed with occupations), you can fit a multilevel model to account for the lack of independence within these groups. Fit models for continuous, binary, count, ordinal, and survival outcomes. Estimate variances of random intercepts and random coefficients. Compute intraclass correlations. Predict random effects. Estimate relationships that are population averaged over the random effects.
Bayesian analysis
Fit Bayesian regression models using one of the Markov chain Monte Carlo (MCMC) methods. You can choose from various supported models or even program your own. Extensive tools are available to check convergence, including multiple chains. Compute posterior mean estimates and credible intervals for model parameters and functions of model parameters. You can perform both interval- and model-based hypothesis testing. Compare models using Bayes factors. Compute model fit using posterior predictive values and generate predictions. If you want to account for model uncertainty in your regression model, use Bayesian model averaging.
Additive models of relative risk
Determine how exposures interact to put subjects at a higher risk of experiencing an outcome of interest. For example, you might be investigating how exposure to cigarette smoke and asbestos interact to increase the risk of lung cancer. With Stata's reri command, you can measure two–way interactions in an additive model of relative risk, while accounting for other risk factors. Choose from various supported models, such as binomial generalized linear, Poisson, negative binomial, logistic, Cox, parametric survival, and interval–censored parametric and semiparametric survival models. Estimate the relative excess risk due to interaction (RERI), attributable proportion (AP), and synergy index (SI).
Automated reporting and customizable tables
Stata is designed for reproducible research, including the ability to create dynamic documents incorporating your analysis results. Create Word or PDF files, populate Excel worksheets with results and format them to your liking, and mix Markdown, HTML, Stata results, and Stata graphs, all from within Stata. Create tables that compare regression results or summary statistics, use default styles or apply your own, and export your tables to Word, PDF, HTML, LaTeX, Excel, or Markdown and include them in your reports.
Jupyter Notebook with Stata
Jupyter Notebook is widely used by researchers and scientists to share their ideas and results for collaboration and innovation. It is an easy-to-use web application that allows you to combine code, visualizations, mathematical formulas, narrative text, and other rich media in a single document (a "notebook") for interactive computing and developing. You can invoke Stata and Mata from Jupyter Notebook with the IPython (interactive Python) kernel. This means you can combine the capabilities of both Python and Stata in a single environment to make your work easily reproducible and shareable with others.
Features for Biostatisticians
Survival analysis
Analyze duration outcomes—outcomes measuring the time to an event such as failure or death—using Stata's specialized tools for survival analysis. Account for the complications inherent in survival data, such as sometimes not observing the event (right-, left-, and interval-censoring), individuals entering the study at differing times (delayed entry), and individuals who are not continuously observed throughout the study (gaps). You can estimate and plot the probability of survival over time. Or model survival as a function of covariates using Cox, Weibull, lognormal, and other regression models. Predict hazard ratios, mean survival time, and survival probabilities. Do you have groups of individuals in your study? Adjust for within-group correlation with a random-effects or shared-frailty model. If you have many potential covariates, use lasso cox and elasticnet cox for model selection and prediction.
Multilevel mixed-effects models
Whether the groupings in your data arise in a nested fashion (patients nested in clinics and clinics nested in regions) or in a nonnested fashion (regions crossed with occupations), you can fit a multilevel model to account for the lack of independence within these groups. Fit models for continuous, binary, count, ordinal, and survival outcomes. Estimate variances of random intercepts and random coefficients. Compute intraclass correlations. Predict random effects. Estimate relationships that are population averaged over the random effects.
Bayesian analysis
Fit Bayesian regression models using one of the Markov chain Monte Carlo (MCMC) methods. You can choose from various supported models or even program your own. Extensive tools are available to check convergence, including multiple chains. Compute posterior mean estimates and credible intervals for model parameters and functions of model parameters. You can perform both interval- and model-based hypothesis testing. Compare models using Bayes factors. Compute model fit using posterior predictive values and generate predictions. If you want to account for model uncertainty in your regression model, use Bayesian model averaging.
Power, precision and sample size
Before you conduct your experiment, determine the sample size needed to detect meaningful effects without wasting resources. Do you intend to compute CIs for means or variances or perform tests for proportions or correlations? Do you plan to fit a Cox proportional hazards model or compare survivor functions using a log-rank test? Do you want to use a Cochran—Mantel—Haenszel test of association or a Cochran—Armitage trend test? Use Stata's power command to compute power and sample size, create customized tables, and automatically graph the relationships between power, sample size, and effect size for your planned study. Or use the ciwidth command to do the same but for CIs instead of hypothesis tests by computing the required sample size for the desired CI precision. Or use gsdesign to compute stopping boundaries and the required sample sizes for group sequential designs. Instead of commands, use the interactive Control Panel to perform your analysis.
Linear, binary and count regressions
Fit classical ANOVA and linear regression models of the relationship between a continuous outcome, such as weight, and the determinants of weight, such as height, diet, and level of exercise. If your response is binary, ordinal, categorical, or count, don't worry. Stata has estimators for these types of outcomes too. Use logistic regression to estimate odds ratios. Estimate incidence rates using a Poisson model. Analyze matched case–control data with conditional logistic regression. A vast array of tools is available after fitting such models. Predict outcomes and their confidence intervals. Test equality of parameters. Compute linear and nonlinear combinations of parameters.
Meta-analysis
Combine results of multiple studies to estimate an overall effect. Use forest plots to visualize results. Use subgroup analysis and meta-regression to explore study heterogeneity. Use funnel plots and formal tests to explore publication bias and small-study effects. Use trim-and-fill analysis to assess the impact of publication bias on results. Perform cumulative and leave-one-out meta-analysis. Perform univariate, multilevel, and multivariate meta-analysis. Use the meta suite, or let the Control Panel interface guide you through your entire meta-analysis.
Multiple imputation
Account for missing data in your sample using multiple imputation. Choose from univariate and multivariate methods to impute missing values in continuous, censored, truncated, binary, ordinal, categorical, and count variables. Then, in a single step, estimate parameters using the imputed datasets, and combine results. Fit a linear model, logit model, Poisson model, hierarchical model, survival model, or one of the many other supported models. Use the mi command, or let the Control Panel interface guide you through your entire MI analysis.
Marginal means, contrasts and interactions
Marginal means and contrasts let you analyze the relationships between your outcome variable and your covariates, even when that outcome is binary, count, ordinal, categorical, or survival. Compute adjusted predictions with covariates set to interesting or representative values. Or compute marginal means for each level of a categorical covariate. Make comparisons of the adjusted predictions or marginal means using contrasts. If you have multilevel data and random effects, these effects are automatically integrated out to provide marginal (that is, population-averaged) estimates. After fitting almost any model in Stata, analyze the effect of covariate interactions, and easily create plots to visualize those interactions.
Causal inference
Estimate experimental-style causal effects from observational data. With Stata's treatment-effects estimators, you can use a potential-outcomes (counterfactuals) framework to estimate, for instance, the effect of family structure on child development or the effect of unemployment on anxiety. Fit models for continuous, binary, count, fractional, and survival outcomes with binary or multivalued treatments using inverse-probability weighting (IPW), propensity-score matching, nearest-neighbor matching, regression adjustment, or doubly robust estimators. If the assignment to a treatment is not independent of the outcome, you can use an endogenous treatment-effects estimator. In the presence of group and time effects, you can use difference-in-differences (DID) and triple-differences (DDD) estimators. In the presence of high-dimensional covariates, you can use lasso. If causal effects are mediated through another variable, use causal mediation with mediate to disentangle direct and indirect effects.
Epidemiological tables
Want to analyze data from a prospective (incidence) study, cohort study, case–control study, or matched case–control study? Stata's tables for epidemiologists make it easy to summarize your data and compute statistics such as incidence-rate ratios, incidence-rate differences, risk ratios, risk differences, odds ratios, and attributable fractions. You can analyze stratified data too—compute Mantel–Haenszel combined estimates, perform tests of homogeneity, and standardize estimates. If you have an ordinal rather than binary exposure, you can perform a test for a trend.
Programming
Want to program your own commands to perform estimation, perform data management, or implement other new features? Stata is programmable, and thousands of Stata users have implemented and published thousands of community-contributed commands. These commands look and act just like official Stata commands and are easily installed for free over the Internet from within Stata. A unique feature of Stata's programming environment is Mata, a fast and compiled language with support for matrix types. Of course, it has all the advanced matrix operations you need. It also has access to the power of LAPACK. What's more, it has built-in solvers and optimizers to make implementing your own maximum likelihood, GMM, or other estimators easier. And you can leverage all of Stata's estimation and other features from within Mata. Many of Stata's official commands are themselves implemented in Mata.
PyStata - Python integration
Interact Stata code with Python code. You can seamlessly pass data and results between Stata and Python. You can use Stata within Jupyter Notebook and other IPython environments. You can call Python libraries such as NumPy, matplotlib, Scrapy, scikit-learn, and more from Stata. You can use Stata analyses from within Python.
Automated reporting and customizable tables
Stata is designed for reproducible research, including the ability to create dynamic documents incorporating your analysis results. Create Word or PDF files, populate Excel worksheets with results and format them to your liking, and mix Markdown, HTML, Stata results, and Stata graphs, all from within Stata. Create tables that compare regression results or summary statistics, use default styles or apply your own, and export your tables to Word, PDF, HTML, LaTeX, Excel, or Markdown and include them in your reports.
Features for Medical Researchers
General linear models
Fit one- and two-way models. Or fit models with three, four, or even more factors. Analyze data with nested factors, with fixed and random factors, or with repeated measures. Use ANCOVA models when you have continuous covariates and MANOVA models when you have multiple outcome variables. Further explore the relationships between your outcome and predictors by estimating effect sizes and computing least-squares and marginal means. Perform contrasts and pairwise comparisons. Analyze and plot interactions.
Linear, binary and count regressions
Fit classical ANOVA and linear regression models of the relationship between a continuous outcome, such as weight, and the determinants of weight, such as height, diet, and level of exercise. If your response is binary, ordinal, categorical, or count, don't worry. Stata has estimators for these types of outcomes too. Use logistic regression to estimate odds ratios. Estimate incidence rates using a Poisson model. Analyze matched case–control data with conditional logistic regression. A vast array of tools is available after fitting such models. Predict outcomes and their confidence intervals. Test equality of parameters. Compute linear and nonlinear combinations of parameters.
Power, precision and sample size
Before you conduct your experiment, determine the sample size needed to detect meaningful effects without wasting resources. Do you intend to compute CIs for means or variances or perform tests for proportions or correlations? Do you plan to fit a Cox proportional hazards model or compare survivor functions using a log-rank test? Do you want to use a Cochran—Mantel—Haenszel test of association or a Cochran—Armitage trend test? Use Stata's power command to compute power and sample size, create customized tables, and automatically graph the relationships between power, sample size, and effect size for your planned study. Or use the ciwidth command to do the same but for CIs instead of hypothesis tests by computing the required sample size for the desired CI precision. Or use gsdesign to compute stopping boundaries and the required sample sizes for group sequential designs. Instead of commands, use the interactive Control Panel to perform your analysis.
Marginal means, contrasts and interactions
Marginal means and contrasts let you analyze the relationships between your outcome variable and your covariates, even when that outcome is binary, count, ordinal, categorical, or survival. Compute adjusted predictions with covariates set to interesting or representative values. Or compute marginal means for each level of a categorical covariate. Make comparisons of the adjusted predictions or marginal means using contrasts. If you have multilevel data and random effects, these effects are automatically integrated out to provide marginal (that is, population-averaged) estimates. After fitting almost any model in Stata, analyze the effect of covariate interactions, and easily create plots to visualize those interactions.
Multilevel mixed-effects models
Whether the groupings in your data arise in a nested fashion (patients nested in clinics and clinics nested in regions) or in a nonnested fashion (regions crossed with occupations), you can fit a multilevel model to account for the lack of independence within these groups. Fit models for continuous, binary, count, ordinal, and survival outcomes. Estimate variances of random intercepts and random coefficients. Compute intraclass correlations. Predict random effects. Estimate relationships that are population averaged over the random effects.
Meta-analysis
Combine results of multiple studies to estimate an overall effect. Use forest plots to visualize results. Use subgroup analysis and meta-regression to explore study heterogeneity. Use funnel plots and formal tests to explore publication bias and small-study effects. Use trim-and-fill analysis to assess the impact of publication bias on results. Perform cumulative and leave-one-out meta-analysis. Perform univariate, multilevel, and multivariate meta-analysis. Use the meta suite, or let the Control Panel interface guide you through your entire meta-analysis.
Multiple imputation
Account for missing data in your sample using multiple imputation. Choose from univariate and multivariate methods to impute missing values in continuous, censored, truncated, binary, ordinal, categorical, and count variables. Then, in a single step, estimate parameters using the imputed datasets, and combine results. Fit a linear model, logit model, Poisson model, hierarchical model, survival model, or one of the many other supported models. Use the mi command, or let the Control Panel interface guide you through your entire MI analysis.
Survival analysis
Analyze duration outcomes—outcomes measuring the time to an event such as failure or death—using Stata's specialized tools for survival analysis. Account for the complications inherent in survival data, such as sometimes not observing the event (right-, left-, and interval-censoring), individuals entering the study at differing times (delayed entry), and individuals who are not continuously observed throughout the study (gaps). You can estimate and plot the probability of survival over time. Or model survival as a function of covariates using Cox, Weibull, lognormal, and other regression models. Predict hazard ratios, mean survival time, and survival probabilities. Do you have groups of individuals in your study? Adjust for within-group correlation with a random-effects or shared-frailty model. If you have many potential covariates, use lasso cox and elasticnet cox for model selection and prediction.
Epidemiological tables
Want to analyze data from a prospective (incidence) study, cohort study, case–control study, or matched case–control study? Stata's tables for epidemiologists make it easy to summarize your data and compute statistics such as incidence-rate ratios, incidence-rate differences, risk ratios, risk differences, odds ratios, and attributable fractions. You can analyze stratified data too—compute Mantel–Haenszel combined estimates, perform tests of homogeneity, and standardize estimates. If you have an ordinal rather than binary exposure, you can perform a test for a trend.
Additive models of relative risk
Determine how exposures interact to put subjects at a higher risk of experiencing an outcome of interest. For example, you might be investigating how exposure to cigarette smoke and asbestos interact to increase the risk of lung cancer. With Stata's reri command, you can measure two–way interactions in an additive model of relative risk, while accounting for other risk factors. Choose from various supported models, such as binomial generalized linear, Poisson, negative binomial, logistic, Cox, parametric survival, and interval–censored parametric and semiparametric survival models. Estimate the relative excess risk due to interaction (RERI), attributable proportion (AP), and synergy index (SI).
Automated reporting and customizable tables
Stata is designed for reproducible research, including the ability to create dynamic documents incorporating your analysis results. Create Word or PDF files, populate Excel worksheets with results and format them to your liking, and mix Markdown, HTML, Stata results, and Stata graphs, all from within Stata. Create tables that compare regression results or summary statistics, use default styles or apply your own, and export your tables to Word, PDF, HTML, LaTeX, Excel, or Markdown and include them in your reports.
Jupyter Notebook with Stata
Jupyter Notebook is widely used by researchers and scientists to share their ideas and results for collaboration and innovation. It is an easy-to-use web application that allows you to combine code, visualizations, mathematical formulas, narrative text, and other rich media in a single document (a "notebook") for interactive computing and developing. You can invoke Stata and Mata from Jupyter Notebook with the IPython (interactive Python) kernel. This means you can combine the capabilities of both Python and Stata in a single environment to make your work easily reproducible and shareable with others.
Features for Sociologists
Survey methods
Whether your data require a simple weighted adjustment because of differential sampling rates or you have data from a complex multistage survey, Stata's survey features can provide you with correct standard errors and confidence intervals for your inferences. Simply specify the relevant characteristics of your sampling design, such as sampling weights (including weights at multiple stages), clustering (at one, two, or more stages), stratification, and poststratification. After that, most of Stata's estimation commands can adjust their estimates to correct for your sampling design.
Multiple imputation
Account for missing data in your sample using multiple imputation. Choose from univariate and multivariate methods to impute missing values in continuous, censored, truncated, binary, ordinal, categorical, and count variables. Then, in a single step, estimate parameters using the imputed datasets, and combine results. Fit a linear model, logit model, Poisson model, multilevel model, survival model, or one of the many other supported models. Use the mi command, or let the Control Panel interface guide you through your entire MI analysis.
Multilevel mixed-effects models
Whether the groupings in your data arise in a nested fashion (students nested in schools and schools nested in districts) or in a nonnested fashion (regions crossed with occupations), you can fit a multilevel model to account for the lack of independence within these groups. Fit models for continuous, binary, count, ordinal, and survival outcomes. Estimate variances of random intercepts and random coefficients. Compute intraclass correlations. Predict random effects. Estimate relationships that are population averaged over the random effects.
Panel data
Take full advantage of the extra information that panel data provide while simultaneously handling the peculiar difficulties that panel data present. Study the time-invariant idiosyncratic features within each panel, the relationships across panels, and how outcomes of interest change over time. Fit linear models or nonlinear models for binary, count, ordinal, censored, or survival outcomes with fixed-effects, random-effects, or population-averaged estimators. Fit dynamic models or models with endogeneity. Fit Bayesian panel-data models.
Meta-analysis
Combine results of multiple studies to estimate an overall effect. Use forest plots to visualize results. Use subgroup analysis and meta-regression to explore study heterogeneity. Use funnel plots and formal tests to explore publication bias and small-study effects. Use trim-and-fill analysis to assess the impact of publication bias on results. Perform cumulative and leave-one-out meta-analysis. Perform univariate, multilevel, and multivariate meta-analysis. Use the meta suite, or let the Control Panel interface guide you through your entire meta-analysis.
Linear, binary and count regressions
Fit classical linear models of the relationship between a continuous outcome, such as wage, and the determinants of wage, such as education level, age, experience, and economic sector. If your response is binary (for example, employed or unemployed), ordinal (education level), or count (number of children), don't worry. Stata has maximum likelihood estimators—probit, ordered probit, Poisson, and many others—that estimate the relationship between such outcomes and their determinants. A vast array of tools is available to analyze such models. Predict outcomes and their confidence intervals. Test equality of parameters or any linear or nonlinear combination of parameters.
Structural equation modeling (SEM)
Estimate mediation effects, analyze the relationship between an unobserved latent concept such as a person's level of conservatism and the observed variables that measure conservatism, model a system with many endogenous variables and correlated errors, or fit a model with complex relationships among both latent and observed variables. Fit models with continuous, binary, count, ordinal, fractional, and survival outcomes. Even fit multilevel models with groups of correlated observations such as children within the same schools. Evaluate model fit. Compute indirect and total effects. Fit models by drawing a path diagram or using the straightforward command syntax.
Adjusted predictions, interactions and moderation
Adjusted predictions and marginal means let you analyze the relationships between your outcome variable and your covariates, even when that outcome is binary, count, ordinal, or categorical. Compute adjusted predictions with covariates set to interesting or representative values. Or compute marginal means for each level of a categorical covariate. Make comparisons of the adjusted predictions or marginal means using contrasts. If you have multilevel or panel data and random effects, these effects are automatically integrated out to provide marginal (that is, population-averaged) estimates. After fitting almost any model in Stata, analyze the effect of moderating variables, and easily create interaction plots.
Choice Models
Model your discrete choice data. If your outcome is, for instance, high-school graduates' choices to attend college, attend a trade school, or to work, you can fit a conditional logit, multinomial probit, or mixed logit model. Is your outcome instead a ranking of prefered alternatives? Fit a rank-ordered probit or rank-ordered logit model. Regardless of the model fit, you can use the margins to easily interpret the results. Estimate how much distance to the nearest college affects the probability of enrolling in college and even the probability of going to a trade school.
Bayesian analysis
Fit Bayesian regression models using one of the Markov chain Monte Carlo (MCMC) methods. You can choose from various supported models or even program your own. Extensive tools are available to check convergence, including multiple chains. Compute posterior mean estimates and credible intervals for model parameters and functions of model parameters. You can perform both interval- and model-based hypothesis testing. Compare models using Bayes factors. Compute model fit using posterior predictive values and generate predictions. If you want to account for model uncertainty in your regression model, use Bayesian model averaging.
Causal inference
Estimate experimental-style causal effects from observational data. With Stata's treatment-effects estimators, you can use a potential-outcomes (counterfactuals) framework to estimate, for instance, the effect of family structure on child development or the effect of unemployment on anxiety. Fit models for continuous, binary, count, fractional, and survival outcomes with binary or multivalued treatments using inverse-probability weighting (IPW), propensity-score matching, nearest-neighbor matching, regression adjustment, or doubly robust estimators. If the assignment to a treatment is not independent of the outcome, you can use an endogenous treatment-effects estimator. In the presence of group and time effects, you can use difference-in-differences (DID) and triple-differences (DDD) estimators. In the presence of high-dimensional covariates, you can use lasso. If causal effects are mediated through another variable, use causal mediation with mediate to disentangle direct and indirect effects.
Programming
Want to program your own commands to perform estimation, perform data management, or implement other new features? Stata is programmable, and thousands of Stata users have implemented and published thousands of community-contributed commands. These commands look and act just like official Stata commands and are easily installed for free over the Internet from within Stata. A unique feature of Stata's programming environment is Mata, a fast and compiled language with support for matrix types. Of course, it has all the advanced matrix operations you need. It also has access to the power of LAPACK. What's more, it has built-in solvers and optimizers to make implementing your own maximum likelihood, GMM, or other estimators easier. And you can leverage all of Stata's estimation and other features from within Mata. Many of Stata's official commands are themselves implemented in Mata.
Automated reporting and customizable tables
Stata is designed for reproducible research, including the ability to create dynamic documents incorporating your analysis results. Create Word or PDF files, populate Excel worksheets with results and format them to your liking, and mix Markdown, HTML, Stata results, and Stata graphs, all from within Stata. Create tables that compare regression results or summary statistics, use default styles or apply your own, and export your tables to Word, PDF, HTML, LaTeX, Excel, or Markdown and include them in your reports.
Jupyter Notebook with Stata
Jupyter Notebook is widely used by researchers and scientists to share their ideas and results for collaboration and innovation. It is an easy-to-use web application that allows you to combine code, visualizations, mathematical formulas, narrative text, and other rich media in a single document (a "notebook") for interactive computing and developing. You can invoke Stata and Mata from Jupyter Notebook with the IPython (interactive Python) kernel. This means you can combine the capabilities of both Python and Stata in a single environment to make your work easily reproducible and shareable with others.
Features for Public Health Professionals
Survey methods
Whether your data require a simple weighted adjustment because of differential sampling rates or you have data from a complex multistage survey, Stata's survey features can provide you with correct standard errors and confidence intervals for your inferences. Simply specify the relevant characteristics of your sampling design, such as sampling weights (including weights at multiple stages), clustering (at one, two, or more stages), stratification, and poststratification. After that, most of Stata's estimation commands can adjust their estimates to correct for your sampling design.
Multilevel mixed-effects models
Whether the groupings in your data arise in a nested fashion (patients nested in clinics and clinics nested in regions) or in a nonnested fashion (regions crossed with occupations), you can fit a multilevel model to account for the lack of independence within these groups. Fit models for continuous, binary, count, ordinal, and survival outcomes. Estimate variances of random intercepts and random coefficients. Compute intraclass correlations. Predict random effects. Estimate relationships that are population averaged over the random effects.
Panel data
Take full advantage of the extra information that panel data provide while simultaneously handling the peculiarities of panel data. Study the time-invariant features within each panel, the relationships across panels, and how outcomes of interest change over time. Fit linear models or nonlinear models for binary, count, ordinal, censored, or survival outcomes with fixed-effects, random-effects, or population-averaged estimators. Fit dynamic models or models with endogeneity. Fit Bayesian panel-data models.
Structural equation modeling (SEM)
Estimate mediation effects, analyze the relationship between an unobserved latent concept such as depression and the observed variables that measure depression, model a system with many endogenous variables and correlated errors, or fit a model with complex relationships among both latent and observed variables. Fit models with continuous, binary, count, ordinal, fractional, and survival outcomes. Even fit multilevel models with groups of correlated observations such as children within the same schools. Evaluate model fit. Compute indirect and total effects. Fit models by drawing a path diagram or using the straightforward command syntax.
Linear, binary and count regressions
Fit classical linear models of the relationship between a continuous outcome, such as wage, and the determinants of wage, such as education level, age, experience, and economic sector. If your response is binary (for example, employed or unemployed), ordinal (education level), or count (number of children), don't worry. Stata has maximum likelihood estimators—probit, ordered probit, Poisson, and many others—that estimate the relationship between such outcomes and their determinants. A vast array of tools is available to analyze such models. Predict outcomes and their confidence intervals. Test equality of parameters or any linear or nonlinear combination of parameters.
Meta-analysis
Combine results of multiple studies to estimate an overall effect. Use forest plots to visualize results. Use subgroup analysis and meta-regression to explore study heterogeneity. Use funnel plots and formal tests to explore publication bias and small-study effects. Use trim-and-fill analysis to assess the impact of publication bias on results. Perform cumulative and leave-one-out meta-analysis. Perform univariate, multilevel, and multivariate meta-analysis. Use the meta suite, or let the Control Panel interface guide you through your entire meta-analysis.
Multiple imputation
Account for missing data in your sample using multiple imputation. Choose from univariate and multivariate methods to impute missing values in continuous, censored, truncated, binary, ordinal, categorical, and count variables. Then, in a single step, estimate parameters using the imputed datasets, and combine results. Fit a linear model, logit model, Poisson model, hierarchical model, survival model, or one of the many other supported models. Use the mi command, or let the Control Panel interface guide you through your entire MI analysis.
Adjusted predictions, contrasts and interactions
Adjusted predictions and contrasts let you analyze the relationships between your outcome variable and your covariates, even when that outcome is binary, count, ordinal, or categorical. Compute adjusted predictions with covariates set to interesting or representative values. Or compute marginal means for each level of a categorical covariate. Make comparisons of the adjusted predictions or marginal means using contrasts. If you have multilevel or panel data and random effects, these effects are automatically integrated out to provide marginal (that is, population-averaged) estimates. After fitting almost any model in Stata, analyze the effect of covariate interactions, and easily create plots to visualize those interactions.
Survival analysis
Analyze duration outcomes—outcomes measuring the time to an event such as failure or death—using Stata's specialized tools for survival analysis. Account for the complications inherent in survival data, such as sometimes not observing the event (right-, left-, and interval-censoring), individuals entering the study at differing times (delayed entry), and individuals who are not continuously observed throughout the study (gaps). You can estimate and plot the probability of survival over time. Or model survival as a function of covariates using Cox, Weibull, lognormal, and other regression models. Predict hazard ratios, mean survival time, and survival probabilities. Do you have groups of individuals in your study? Adjust for within-group correlation with a random-effects or shared-frailty model. If you have many potential covariates, use lasso cox and elasticnet cox for model selection and prediction.
Causal inference
Estimate experimental-style causal effects from observational data. With Stata's treatment-effects estimators, you can use a potential-outcomes (counterfactuals) framework to estimate, for instance, the effect of family structure on child development or the effect of unemployment on anxiety. Fit models for continuous, binary, count, fractional, and survival outcomes with binary or multivalued treatments using inverse-probability weighting (IPW), propensity-score matching, nearest-neighbor matching, regression adjustment, or doubly robust estimators. If the assignment to a treatment is not independent of the outcome, you can use an endogenous treatment-effects estimator. In the presence of group and time effects, you can use difference-in-differences (DID) and triple-differences (DDD) estimators. In the presence of high-dimensional covariates, you can use lasso. If causal effects are mediated through another variable, use causal mediation with mediate to disentangle direct and indirect effects.
Time Series
Handle the statistical challenges inherent to time-series data—autocorrelations, common factors, autoregressive conditional heteroskedasticity, unit roots, cointegration, and much more. Analyze univariate time series using ARIMA, ARFIMA, Markov-switching models, ARCH and GARCH models, and unobserved-components models. Analyze multivariate time series using VAR, structural VAR, VEC, multivariate GARCH, dynamic-factor models, and state-space models. Compute and graph impulse responses. Test for unit roots. Perform Bayesian time-series analysis.
IRT (item response theory)
Explore the relationship between unobserved latent characteristics such as hospital satisfaction and the probability of responding positively to questionnaire items related to satisfaction. Or explore the relationship between unobserved health and self-reported responses to questions about mobility, independence, and other health-affected activities. IRT can be used to create measures of such unobserved traits or place individuals on a scale measuring the trait. It can also be used to select the best items for measuring a latent trait. IRT models are available for binary, graded, rated, partial-credit, and nominal response items. Visualize the relationships using item characteristic curves, and measure overall test performance using test information functions.
Automated reporting and customizable tables
Stata is designed for reproducible research, including the ability to create dynamic documents incorporating your analysis results. Create Word or PDF files, populate Excel worksheets with results and format them to your liking, and mix Markdown, HTML, Stata results, and Stata graphs, all from within Stata. Create tables that compare regression results or summary statistics, use default styles or apply your own, and export your tables to Word, PDF, HTML, LaTeX, Excel, or Markdown and include them in your reports.
Jupyter Notebook with Stata
Jupyter Notebook is widely used by researchers and scientists to share their ideas and results for collaboration and innovation. It is an easy-to-use web application that allows you to combine code, visualizations, mathematical formulas, narrative text, and other rich media in a single document (a "notebook") for interactive computing and developing. You can invoke Stata and Mata from Jupyter Notebook with the IPython (interactive Python) kernel. This means you can combine the capabilities of both Python and Stata in a single environment to make your work easily reproducible and shareable with others.
Privacy Overview
Ce site utilise des cookies pour améliorer votre expérience pendant votre navigation. Parmi ces cookies, ceux classés comme nécessaires sont stockés sur votre navigateur car ils sont essentiels au bon fonctionnement des fonctionnalités de base du site. Nous utilisons également des cookies tiers qui nous aident à analyser et à comprendre comment vous utilisez ce site web. Ces cookies ne seront stockés dans votre navigateur qu’avec votre consentement. Vous avez également la possibilité de refuser ces cookies. Cependant, le refus de certains cookies peut avoir un impact sur votre expérience de navigation.
Essential
Name | Description | Lifetime |
---|---|---|
ADD_TO_CART | (Adobe Commerce only) Used by Google Tag Manager | 1 Year |
GUEST-VIEW | Stores the Order ID that guest shoppers use to retrieve their order status. Guest orders view. Used in Orders and Returns widgets | 1 Year |
LOGIN_REDIRECT | Preserves the destination page that was loading before the customer was directed to log in | 1 Year |
MAGE-BANNERS-CACHE-STORAGE | (Adobe Commerce only) Stores banner content locally to improve performance | 1 Year |
MAGE-MESSAGES | Tracks error messages and other notifications that are shown to the user | 1 Year |
MAGE-TRANSLATION-STORAGE | Stores translated content when requested by the shopper | 1 Year |
MAGE-TRANSLATION-FILE-VERSION | Tracks the version of translations in local storage | 1 Year |
PRODUCT_DATA_STORAGE | Stores configuration for product data related to Recently Viewed/Compared Products | 1 Year |
RECENTLY_COMPARED_PRODUCT | Stores product IDs of recently compared products | 1 Year |
RECENTLY_COMPARED_PRODUCT_PREVIOUS | Stores product IDs of previously compared products for easy navigation | 1 Year |
RECENTLY_VIEWED_PRODUCT | Stores product IDs of recently viewed products for easy navigation | 1 Year |
RECENTLY_VIEWED_PRODUCT_PREVIOUS | Stores product IDs of recently previously viewed products for easy navigation | 1 Year |
REMOVE_FROM_CART | (Adobe Commerce only) Used by Google Tag Manager | 1 Year |
STF | Records the time messages are sent by the SendFriend | 1 Year |
X-MAGENTO-VARY | Configuration setting that improves performance when using Varnish static content caching | 1 Year |
FORM_KEY | A security measure that appends a random string to all form submissions to protect the data from Cross-Site Request Forgery | 1 Year |
MAGE-CACHE-SESSID | The value of this cookie triggers the cleanup of local cache storage | 1 Year |
MAGE-CACHE-STORAGE | Local storage of visitor-specific content that enables ecommerce functions | 1 Year |
MAGE-CACHE-STORAGE-SECTION-INVALIDATION | Forces local storage of specific content sections that should be invalidated | 1 Year |
PERSISTENT_SHOPPING_CART | Stores the key (ID) of persistent cart to make it possible to restore the cart for an anonymous shopper | 1 Year |
PRIVATE_CONTENT_VERSION | Appends a random, unique number and time to pages with customer content to prevent them from being cached on the server | 1 Year |
SECTION_DATA_IDS | Stores customer-specific information related to shopper-initiated actions, such as wish list display and checkout information | 1 Year |
STORE | Tracks the specific store view/locale selected by the shopper | 1 Year |
Marketing
Name | Description | Lifetime |
---|---|---|
CUSTOMER_SEGMENT_IDS | Stores your Customer Segment ID | 1 Year |
EXTERNAL_NO_CACHE | A flag that, indicates whether caching is on or off | 1 Year |
FRONTEND | Your session ID on the server | 1 Year |
GUEST-VIEW | Allows guests to edit their orders | 1 Year |
LAST_CATEGORY | The last category you visited | 1 Year |
LAST_PRODUCT | The last product you looked at | 1 Year |
NEWMESSAGE | Indicates whether a new message has been received | 1 Year |
NO_CACHE | Indicates whether it is allowed to use cache | 1 Year |
Functionality
Name | Description | Lifetime |
---|---|---|
MG_DNT | Allows you to restrict Adobe Commerce data collection if you have custom code to manage cookie consent on your site | 1 Year |
USER_ALLOWED_SAVE_COOKIE | Used for cookie restriction mode | 1 Year |
AUTHENTICATION_FLAG | Indicates if a shopper has signed in or signed out | 1 Year |
DATASERVICES_CUSTOMER_ID | Indicates if a shopper has signed in or signed out | 1 Year |
DATASERVICES_CUSTOMER_GROUP | Indicates a customer's group. This cookie is stored as sha1 checksum of the customer's group ID | 1 Year |
DATASERVICES_CART_ID | Identifies a shopper's cart actions | 1 Year |
DATASERVICES_PRODUCT_CONTEXT | Identifies a shopper's product interactions. This cookie contains the customer's unique quote ID in the system | 1 Year |
Statistical
Name | Description | Lifetime |
---|---|---|
_ga | Used by Google Analytics | 1 Year |
_ga_* | Used by Google Analytics | 1 Year |
Validate your login
Se connecter
Créer un nouveau compte